An affective classification method based on deep forest and transfer learning
A sentiment classification and transfer learning technology, applied in the field of image processing, can solve the problems that it is difficult to adjust each parameter to the appropriate value, affect the classification accuracy and speed, and have too many parameters, so as to improve the classification efficiency, reduce the classification cost and high cost. Effect
- Summary
- Abstract
- Description
- Claims
- Application Information
AI Technical Summary
Problems solved by technology
Method used
Image
Examples
Embodiment Construction
[0028] In order to have a clearer understanding of the technical features, purposes and effects of the present invention, the specific implementation manners of the present invention will now be described in detail with reference to the accompanying drawings.
[0029] Embodiments of the present invention provide a sentiment classification method based on deep forest and transfer learning.
[0030] Please refer to figure 1 with figure 2 , figure 1 It is a flowchart of a sentiment classification method based on deep forest and transfer learning in an embodiment of the present invention, figure 2 It is a schematic diagram of the human face emotion classification framework in the embodiment of the present invention, a method of emotion classification based on deep forest and transfer learning, specifically including the following steps:
[0031] S101: Select a source domain data set and a training target domain data set; the source domain data set is a face data set or an emo...
PUM
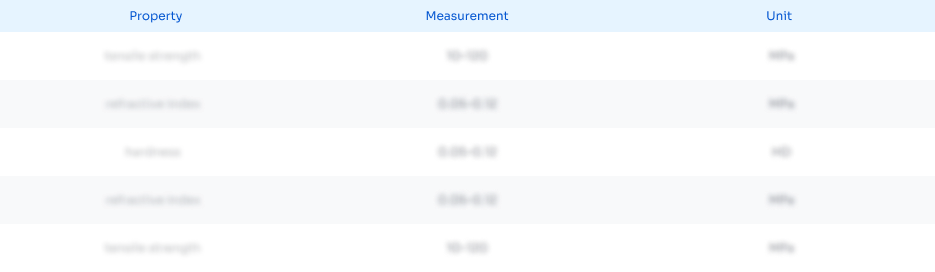
Abstract
Description
Claims
Application Information
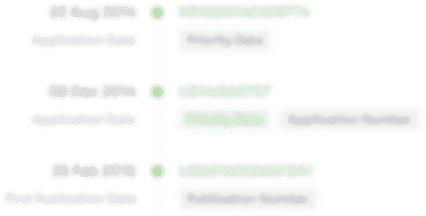
- R&D Engineer
- R&D Manager
- IP Professional
- Industry Leading Data Capabilities
- Powerful AI technology
- Patent DNA Extraction
Browse by: Latest US Patents, China's latest patents, Technical Efficacy Thesaurus, Application Domain, Technology Topic.
© 2024 PatSnap. All rights reserved.Legal|Privacy policy|Modern Slavery Act Transparency Statement|Sitemap