An image super-resolution algorithm based on context-dependent multi-task depth learning
A deep learning, multi-task technology, applied in the field of image super-resolution algorithm, can solve the problems of inconsistency in structure, difficult for neural network to capture structural changes, unreal image details, etc., to achieve the effect of structure preservation
- Summary
- Abstract
- Description
- Claims
- Application Information
AI Technical Summary
Problems solved by technology
Method used
Image
Examples
Embodiment 1
[0033] A context-dependent multi-task deep learning method for static image super-resolution, comprising the following steps:
[0034] S1: collecting image data;
[0035] S2: Establish a neural network model;
[0036] S3: using the collected image data to train the established neural network model;
[0037] S4: Process the trained neural network on static low-resolution images to obtain high-resolution images.
[0038] In step S1, the training data consists of three parts, namely HR images, edge images corresponding to the HR images, and LR images corresponding to the HR images. Wherein, the edge image corresponding to the HR image is a binary image, which can be given by an edge detection algorithm, or can be given by manual marking. Pixels with a value of 0 in the edge image represent non-edges, and pixels with a value of 1 represent edges. Assuming that the resolution of the LR image is h×w and the resolution of the HR image is H×W, the trained network can only be used ...
PUM
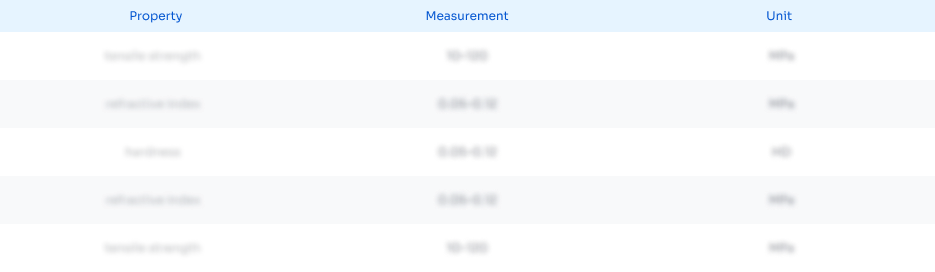
Abstract
Description
Claims
Application Information
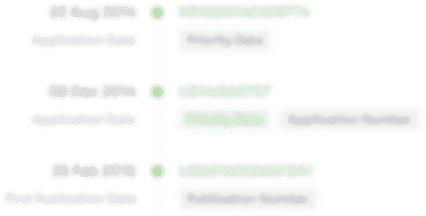
- R&D Engineer
- R&D Manager
- IP Professional
- Industry Leading Data Capabilities
- Powerful AI technology
- Patent DNA Extraction
Browse by: Latest US Patents, China's latest patents, Technical Efficacy Thesaurus, Application Domain, Technology Topic, Popular Technical Reports.
© 2024 PatSnap. All rights reserved.Legal|Privacy policy|Modern Slavery Act Transparency Statement|Sitemap|About US| Contact US: help@patsnap.com