Residual life prediction method of complex equipment based on two-layer long-short-term memory network
A long-short-term memory and life prediction technology, applied in prediction, neural learning methods, biological neural network models, etc., can solve problems such as large amount of data, difficult mapping of algorithms, poor prediction effect, etc., to reduce maintenance costs, Avoid learning, the effect of predictive accuracy improvement
- Summary
- Abstract
- Description
- Claims
- Application Information
AI Technical Summary
Problems solved by technology
Method used
Image
Examples
Embodiment Construction
[0044] The present invention provides a method for predicting the remaining life of complex equipment based on a double-layer long-short-term memory network, which applies a deep learning algorithm to the prediction of the remaining life of complex equipment, and is more conducive to establishing complex equipment-related data and complex equipment remaining life mapping relationship between them. By providing an accurate and reliable prediction method for the remaining life of complex equipment, complex equipment can be maintained in a timely manner, and unnecessary maintenance is reduced, making maintenance of complex equipment targeted, while accurate prediction of the remaining life of complex equipment reduces equipment costs. ACCIDENT.
[0045] seefigure 1 , a method for predicting the remaining life of complex equipment based on a double-layer long-short-term memory network of the present invention, in a specific embodiment, based on the prediction of the remaining life...
PUM
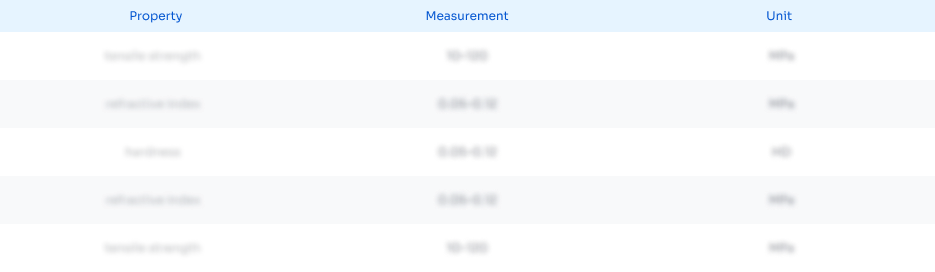
Abstract
Description
Claims
Application Information
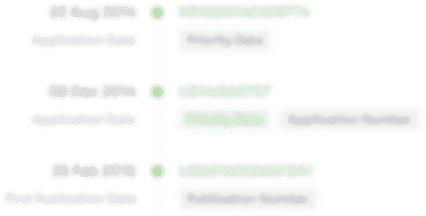
- R&D Engineer
- R&D Manager
- IP Professional
- Industry Leading Data Capabilities
- Powerful AI technology
- Patent DNA Extraction
Browse by: Latest US Patents, China's latest patents, Technical Efficacy Thesaurus, Application Domain, Technology Topic, Popular Technical Reports.
© 2024 PatSnap. All rights reserved.Legal|Privacy policy|Modern Slavery Act Transparency Statement|Sitemap|About US| Contact US: help@patsnap.com