Real-time fault detection and real-time fault isolation of multi-dimensional signals
A real-time fault detection method technology, applied in the field of sensor detection, to achieve the effect of real-time fault detection and real-time fault isolation
- Summary
- Abstract
- Description
- Claims
- Application Information
AI Technical Summary
Problems solved by technology
Method used
Image
Examples
specific Embodiment approach 1
[0026] Specific implementation mode one: the following combination figure 1 This embodiment is described. The real-time fault detection method for multi-dimensional signals described in this embodiment extracts the approximate basis of the normal training set to form the approximate basis of the training samples in the normal state, and uses the approximate basis of the training samples in the normal state to construct the core. Component analysis fault detection model, kernel principal component analysis fault detection model When a fault is detected, a reconstruction-based contribution method is used to detect the locations of all faulty gas sensors.
[0027] In this embodiment, the Kernel Principal Component Analysis (KPCA) method is projected from the original data space to the high-dimensional space F, and the Principal Component Analysis (PCA) method is executed in F.
specific Embodiment approach 2
[0028] Embodiment 2: In this embodiment, Embodiment 1 is further explained. The approximate basis for extracting the normal training set is to use the minimum number of training samples to represent the features of the entire training sample set.
specific Embodiment approach 3
[0029] Specific embodiment three: this embodiment will further explain embodiment one or two, extract the approximate base of the normal training set, form the approximate base of the training sample under the normal state, and use the approximate base of the training sample under the normal state to construct the core The specific process of component analysis fault detection model is as follows:
[0030] X={x n}(n=1,2,...,N) represents the entire training sample set of kernel principal component analysis, where N represents the number of training samples;
[0031] is the entire training sample set X={x n}(n=1,2,…,N), where p(pB the sample size;
[0032] represents the approximate base X B Projection vector in high-dimensional space F;
[0033] per sample x n The projection vector of The approximate value of is expressed as: where θ n =(θ 1 ,θ 2 ,…,θ p ); p Represents the mapping value of the pth training sample in the high-dimensional space F; the projectio...
PUM
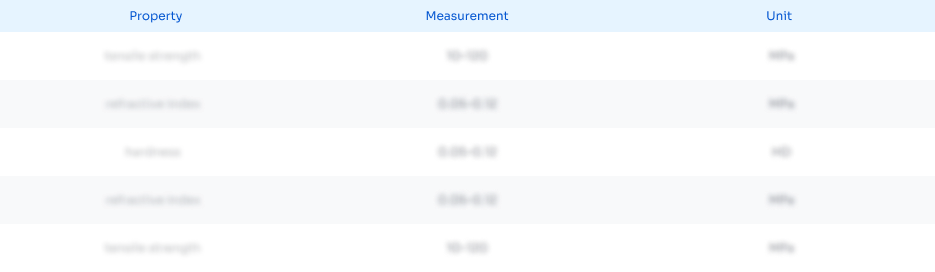
Abstract
Description
Claims
Application Information
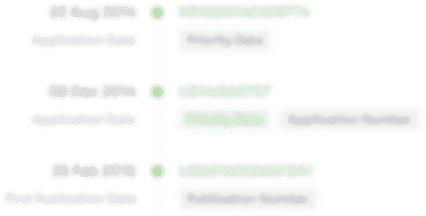
- R&D Engineer
- R&D Manager
- IP Professional
- Industry Leading Data Capabilities
- Powerful AI technology
- Patent DNA Extraction
Browse by: Latest US Patents, China's latest patents, Technical Efficacy Thesaurus, Application Domain, Technology Topic.
© 2024 PatSnap. All rights reserved.Legal|Privacy policy|Modern Slavery Act Transparency Statement|Sitemap