A method for generating a reservoir optimal dispatching decision model based on depth learning
A decision-making model and deep learning technology, applied in instruments, data processing applications, forecasting, etc., can solve the problems of limiting the practical application of the model, limited learning ability, and insufficient attention to time-consuming calculation, so as to enhance practical applicability and ensure simulation. Accuracy and the effect of improving learning ability
- Summary
- Abstract
- Description
- Claims
- Application Information
AI Technical Summary
Problems solved by technology
Method used
Image
Examples
Embodiment Construction
[0034] The present invention will be specifically introduced below in conjunction with the accompanying drawings and specific embodiments.
[0035] Such as figure 1 As shown, the deep learning-based reservoir optimal scheduling decision-making model generation method provided by the embodiment of the present invention includes the following steps:
[0036] S101. Collect historical reservoir scheduling data and reservoir site meteorological data and use deep learning algorithms to obtain historical reservoir scheduling rules.
[0037] S102. According to the historical scheduling data of the reservoir and the meteorological data of the station in the reservoir area, construct a data set including a decision variable Y and an impact factor X, wherein the decision variable includes the outflow Q of each time period of the reservoir O T , the impact factors include: month M, time T, reservoir current period inflow Q i T , the outflow Q of the reservoir in the previous period O...
PUM
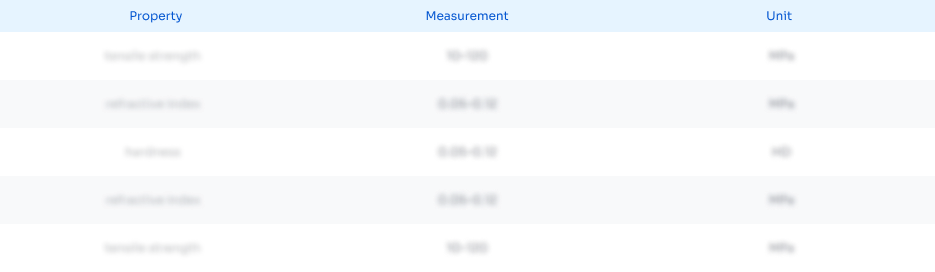
Abstract
Description
Claims
Application Information
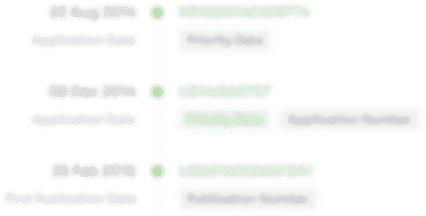
- Generate Ideas
- Intellectual Property
- Life Sciences
- Materials
- Tech Scout
- Unparalleled Data Quality
- Higher Quality Content
- 60% Fewer Hallucinations
Browse by: Latest US Patents, China's latest patents, Technical Efficacy Thesaurus, Application Domain, Technology Topic, Popular Technical Reports.
© 2025 PatSnap. All rights reserved.Legal|Privacy policy|Modern Slavery Act Transparency Statement|Sitemap|About US| Contact US: help@patsnap.com