A fashion compatibility prediction method based on low-rank regularization feature enhancement representation
A technology of feature enhancement and prediction method, applied in the field of clothing analysis in a multimedia environment
- Summary
- Abstract
- Description
- Claims
- Application Information
AI Technical Summary
Problems solved by technology
Method used
Image
Examples
Embodiment 1
[0036] An embodiment of the present invention provides a method for predicting fashion compatibility based on low-rank regularized feature-enhanced representation, see figure 1 , the method includes the following steps:
[0037] 101: Decompose the feature matrix into a first objective function consisting of main features of multiple perspectives and a sparse error matrix;
[0038] 102: Use hypergraph items to standardize the features learned in low-rank subspaces, and obtain the second objective function of the relationship between fashion items:
[0039] 103: Introduce the Grassmannian manifold to obtain the third objective function with the largest distance between the dictionary base matrices under different perspectives;
[0040] 104: Establish the relationship between the characteristics of the multi-view low-rank subspace and the output collocation score, add a sparse regularization term to the least squares loss part, and obtain the typical Lasso regression, which is t...
Embodiment 2
[0048] The scheme in embodiment 1 is further introduced below in conjunction with calculation formula and examples, see the following description for details:
[0049] 201: Using the penultimate fully connected layer of VGGNet19 to extract 1000-D high-level semantic features, plus extracting four kinds of visual features, using the l2 norm to normalize each type of feature, and then concatenating them to form a 1634- The feature representation of D, and finally, the 1634-D feature is normalized to obtain the feature matrix X;
[0050] Among them, the four visual features are: 1) 225-D block color moment, 2) 73-D edge direction histogram, 3) 128-D wavelet texture, 4) 64-D color histogram and 144-D color auto Related graphs.
[0051] 202: Decompose the feature matrix X into main feature parts of multiple perspectives and a sparse error matrix, see the following formula (1);
[0052] Through the processing of step 202, the learned feature matrix has the lowest rank, and the dic...
Embodiment 3
[0078] Provide the test experiment of a kind of fashion compatibility scoring method of the embodiment of the present invention below:
[0079] The detection performance of the embodiment of the present invention is measured by the normalized mean square error (nMSE) and the standard mean square error (rMSE) between the predicted scoring and the real value, defined as follows:
[0080]
[0081]
[0082] in, is the prediction score obtained by the model on the test set, y i is the true value on the test set, and N is the number of samples in the test set.
[0083] In order to evaluate the algorithm performance of this method, the embodiment of the present invention uses 21,889 sets of clothing collocations from the online fashion website Polyvore as a data set, which contains a total of 164,379 fashion items. 17116 outfits are taken for training and 3076 for testing. Take up to 5 pictures for each outfit, such as tops, pants, shoes and accessories. The ratio of the n...
PUM
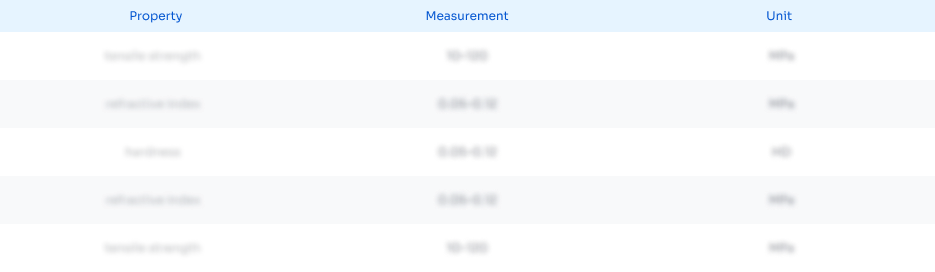
Abstract
Description
Claims
Application Information
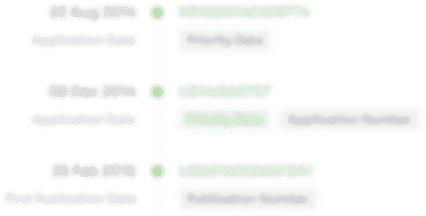
- R&D
- Intellectual Property
- Life Sciences
- Materials
- Tech Scout
- Unparalleled Data Quality
- Higher Quality Content
- 60% Fewer Hallucinations
Browse by: Latest US Patents, China's latest patents, Technical Efficacy Thesaurus, Application Domain, Technology Topic, Popular Technical Reports.
© 2025 PatSnap. All rights reserved.Legal|Privacy policy|Modern Slavery Act Transparency Statement|Sitemap|About US| Contact US: help@patsnap.com