Unsupervised pedestrian re-identification method based on three-data-set cross transfer learning
A pedestrian re-identification and transfer learning technology, applied in the field of unsupervised pedestrian re-identification, can solve the problems of not being able to improve the recognition performance, achieve the effect of improving the re-identification effect, improving the accuracy and robustness, and improving the recognition accuracy
- Summary
- Abstract
- Description
- Claims
- Application Information
AI Technical Summary
Problems solved by technology
Method used
Image
Examples
Embodiment 1
[0044] Such as figure 1 As shown, an unsupervised pedestrian re-identification method based on cross-transfer learning of three data sets, the method includes the following steps:
[0045] When training:
[0046] Step 1: Train three CNNs on large datasets for image classification to obtain three pre-trained models; use these three pre-trained CNNs on three labeled source pedestrian datasets A, B, and C Fine-tuning is performed on the above, so that it can effectively extract pedestrian features;
[0047] Step 2: Use the fine-tuned three CNNs to extract the features of the unlabeled pedestrian pictures in the target data set, and use the K-nearest neighbor regression algorithm to cluster the extracted features respectively;
[0048] Step 3: Screen out the image samples that are close to the center of the cluster after the three models are clustered, and label these samples respectively;
[0049] Step 4: Cross-rotate the sample data labeled with quasi-labels of the three mode...
PUM
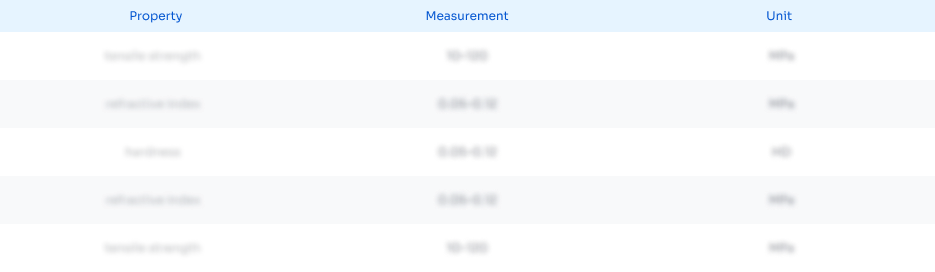
Abstract
Description
Claims
Application Information
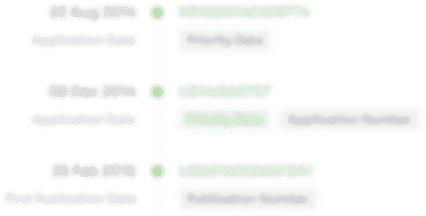
- R&D Engineer
- R&D Manager
- IP Professional
- Industry Leading Data Capabilities
- Powerful AI technology
- Patent DNA Extraction
Browse by: Latest US Patents, China's latest patents, Technical Efficacy Thesaurus, Application Domain, Technology Topic.
© 2024 PatSnap. All rights reserved.Legal|Privacy policy|Modern Slavery Act Transparency Statement|Sitemap