A face synthesis method based on a generative adversarial network
A face synthesis and network technology, applied in biological neural network models, neural learning methods, instruments, etc., can solve problems such as blurred image quality, easy imbalance of multiple networks, and errors in synthetic images
- Summary
- Abstract
- Description
- Claims
- Application Information
AI Technical Summary
Problems solved by technology
Method used
Image
Examples
Embodiment 1
[0070] Figure 1~4 As shown, a face synthesis method based on generating a deep network, including constructing and training an optimized TTGAN model, the TTGAN model is composed of two GAN networks interacting with each other, and the multi-level sparse expression model is consistent with the three transformations Constraints to build a model loss item; then use the trained and optimized TTGAN model to perform face synthesis steps, where the steps to train the TTGAN model are as follows:
[0071] The TTGAN model is composed of two generative confrontation networks with the same structure but opposite face synthesis tasks, which are combined through a loop interaction. Each generative confrontation network GAN is also divided into a generator G and a discriminator D matching combination. The task of the generator is to synthesize faces, and the task of the discriminator is to distinguish between real and synthetic faces. The generator of TTGAN adopts the U-net structure of th...
Embodiment 2
[0123] This embodiment compares the present invention with prior art Pix2Pix GAN and CycleGAN:
[0124] For an objective and fair comparison, this experiment keeps the common basic structure of TTGAN and CycleGAN consistent, while only changing the newly proposed and added structure, the Pix2Pix GAN structure and hyperparameters will keep the default settings of the model. At the same time, the training data set and test set, as well as the number of training times, keep each model consistent.
[0125] 1) Based on the AR face database, facial expression image synthesis.
[0126] a. Randomly select image pairs of 84 people with expressionless normal faces and smiling faces as the training set, and the corresponding image pairs of the other 16 people as the test set.
[0127] b. Use the training set to train TTGAN, CycleGAN and Pix2Pix GAN.
[0128] c. Use the test set to test TTGAN, CycleGAN and Pix2Pix GAN respectively.
[0129] The comparison of images generated by each mo...
PUM
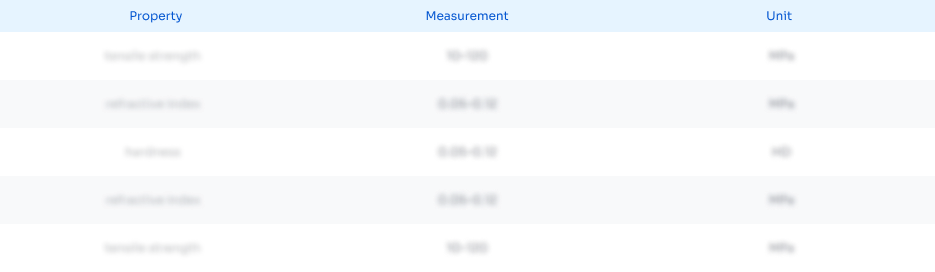
Abstract
Description
Claims
Application Information
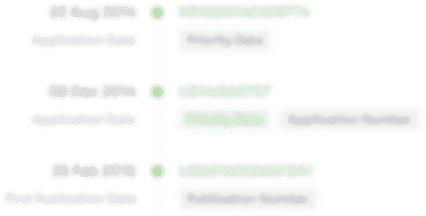
- R&D
- Intellectual Property
- Life Sciences
- Materials
- Tech Scout
- Unparalleled Data Quality
- Higher Quality Content
- 60% Fewer Hallucinations
Browse by: Latest US Patents, China's latest patents, Technical Efficacy Thesaurus, Application Domain, Technology Topic, Popular Technical Reports.
© 2025 PatSnap. All rights reserved.Legal|Privacy policy|Modern Slavery Act Transparency Statement|Sitemap|About US| Contact US: help@patsnap.com