A gas flow data prediction method based on non-equal-length granularity characteristics
A gas flow and data forecasting technology, applied in forecasting, data processing applications, instruments, etc., can solve problems such as large forecasting errors, large scale, and complex gas data input information, so as to reduce the impact of errors, improve accuracy and forecast results The effect of accuracy
- Summary
- Abstract
- Description
- Claims
- Application Information
AI Technical Summary
Problems solved by technology
Method used
Image
Examples
Embodiment Construction
[0015] The technical solutions of the present invention will be further described below in conjunction with specific embodiments and accompanying drawings.
[0016] The present invention proposes a method for predicting gas flow data based on granulation, density clustering and neural network. The specific implementation steps of the method are as follows:
[0017] Step 1: Perform non-equal-length fuzzy granulation based on the time axis for the gas flow collection data;
[0018] Step 1.1: Find the original discrete data change poles, including maximum points and minimum points. During this process, parameters can be modified to control the minimum number of data between each maximum point and each minimum point;
[0019] Step 1.2: According to the difference in the minimum number of data between each maximum value and each minimum value in step 1.1, different granulation methods are used for non-equal-length fuzzy granulation, which is divided into the following two methods; ...
PUM
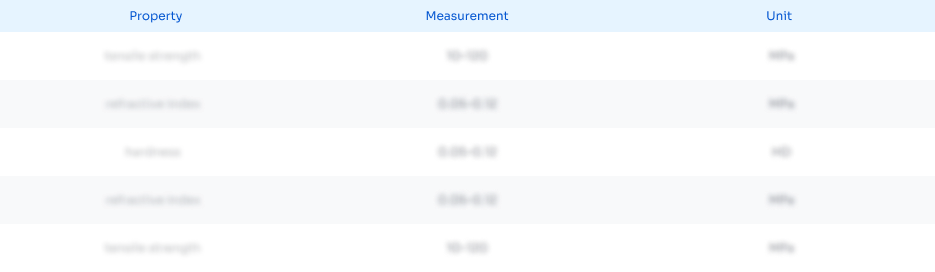
Abstract
Description
Claims
Application Information
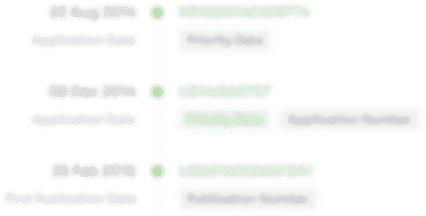
- R&D Engineer
- R&D Manager
- IP Professional
- Industry Leading Data Capabilities
- Powerful AI technology
- Patent DNA Extraction
Browse by: Latest US Patents, China's latest patents, Technical Efficacy Thesaurus, Application Domain, Technology Topic, Popular Technical Reports.
© 2024 PatSnap. All rights reserved.Legal|Privacy policy|Modern Slavery Act Transparency Statement|Sitemap|About US| Contact US: help@patsnap.com