Multi-sensor signal fusion method based on deep learning for gait classification
A multi-sensor and signal fusion technology, applied in the field of biological signal sensing, can solve the problems of inability to obtain complete and comprehensive information of the object, and the data is thin, and achieve the effect of improving the classification effect and improving the accuracy.
- Summary
- Abstract
- Description
- Claims
- Application Information
AI Technical Summary
Problems solved by technology
Method used
Image
Examples
Embodiment Construction
[0039] The present invention will be described in detail below with reference to the accompanying drawings and preferred embodiments.
[0040] The present invention provides a multi-sensor signal fusion method based on deep convolutional neural network for gait classification, the schematic diagram of the method structure is as follows figure 1 shown. This method adopts the following steps to realize:
[0041] Step 1, collecting normal gait information. Fix the IMU and SEMG hardware acquisition system on the outer sides of the left and right calves with straps, where the Y axis of the IMU is perpendicular to the horizontal plane, the X axis is perpendicular to the coronal plane of the human body, and the Z axis is perpendicular to the sagittal plane of the human body. The two channels of SEMG were attached to the gastrocnemius muscle belly and the tibialis anterior muscle belly respectively. The distance between the two electrodes of each channel was 4 cm, and the reference ...
PUM
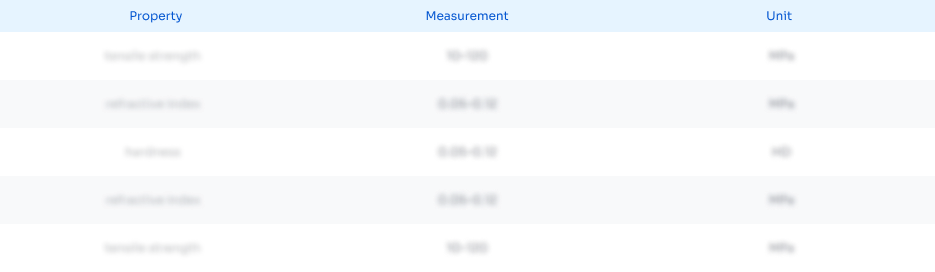
Abstract
Description
Claims
Application Information
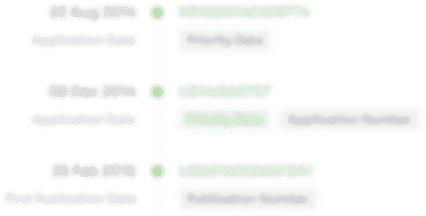
- R&D Engineer
- R&D Manager
- IP Professional
- Industry Leading Data Capabilities
- Powerful AI technology
- Patent DNA Extraction
Browse by: Latest US Patents, China's latest patents, Technical Efficacy Thesaurus, Application Domain, Technology Topic, Popular Technical Reports.
© 2024 PatSnap. All rights reserved.Legal|Privacy policy|Modern Slavery Act Transparency Statement|Sitemap|About US| Contact US: help@patsnap.com