Visual target tracking method based on deep residual network characteristics
A target tracking and network feature technology, applied in the field of visual target tracking based on deep residual network features, can solve problems such as restricting the application of tracking algorithms
- Summary
- Abstract
- Description
- Claims
- Application Information
AI Technical Summary
Problems solved by technology
Method used
Image
Examples
Embodiment Construction
[0037] Such as figure 1 Shown, a kind of visual target tracking method based on depth residual network feature of the present invention, comprises the following steps:
[0038] Step 1. Select the feature layer of the deep residual network and calculate the weight corresponding to each feature layer: In the marked public data set, use each layer in the deep residual network ResNet-N to separately classify the marked public data set Extract features from the video, calculate the tracking overlap rate, select the layer with the top three tracking overlap rates to construct the first training sample, and train the convolutional neural network (CNN) 1 , Convolutional Neural Network CNN 1 by the input layer I 1 , convolutional layer C 1 , pooling layer P 1 , convolutional layer C 2 , pooling layer P 2 , convolutional layer C 3 , pooling layer P 3 , fully connected layer F and output layer O 1 Composed, the image sequence to be tracked passes through the convolutional neural...
PUM
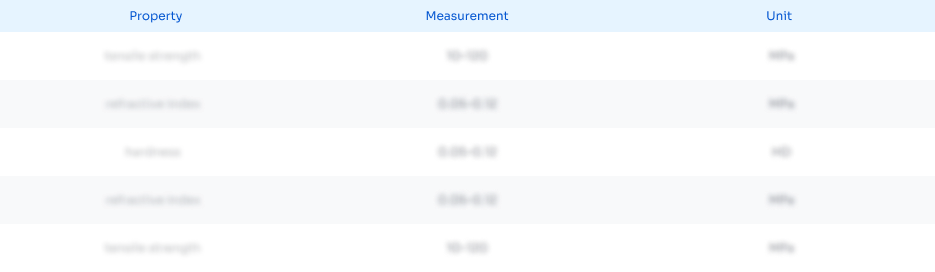
Abstract
Description
Claims
Application Information
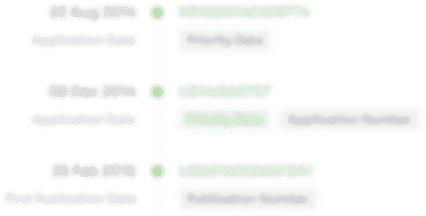
- R&D Engineer
- R&D Manager
- IP Professional
- Industry Leading Data Capabilities
- Powerful AI technology
- Patent DNA Extraction
Browse by: Latest US Patents, China's latest patents, Technical Efficacy Thesaurus, Application Domain, Technology Topic, Popular Technical Reports.
© 2024 PatSnap. All rights reserved.Legal|Privacy policy|Modern Slavery Act Transparency Statement|Sitemap|About US| Contact US: help@patsnap.com