A long short-term memory network (LSTM)-based astronomical big data optically variable curve abnormity detection method
A long-short-term memory and light curve technology, which is applied to biological neural network models, instruments, character and pattern recognition, etc., can solve problems such as difficult processing and large amount of data, achieve short learning time, high precision, and avoid gradient disappearance Effect
- Summary
- Abstract
- Description
- Claims
- Application Information
AI Technical Summary
Problems solved by technology
Method used
Image
Examples
Embodiment Construction
[0023] The implementation process and precautions of the present invention will be further elaborated below. The algorithm is written in python language, importing kears, data analysis package pandas, numerical calculation extension package numpy and matplotlib.pyplot for drawing images.
[0024] S1. Take out the data from the file, do pre-processing, and form a historical time series.
[0025] Historical data is often stored in csv format files. To predict the sequence, the first step is to read the historical data from the file to form a time series. By calling the method in pandas, the historical data can be obtained and the key data, that is, the astronomical light curve data can be extracted.
[0026] Since the data obtained by astronomical telescopes will have different dimensions and serious polarization problems, these problems will cause the learning process to not converge and the desired results will not be obtained. Therefore, the data needs to be normalized. Da...
PUM
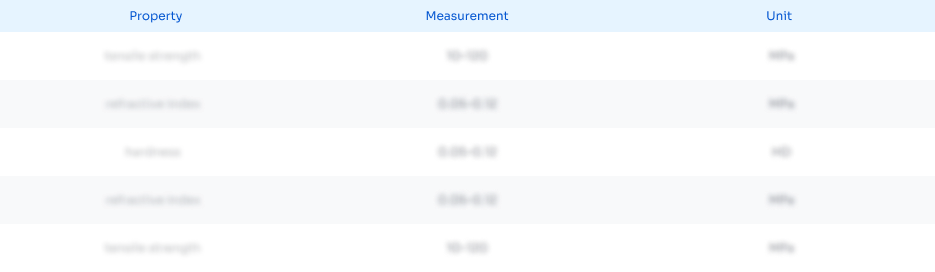
Abstract
Description
Claims
Application Information
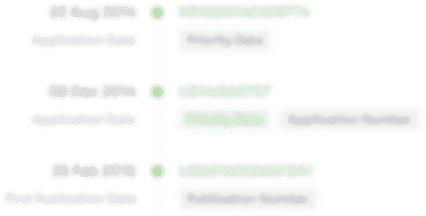
- R&D
- Intellectual Property
- Life Sciences
- Materials
- Tech Scout
- Unparalleled Data Quality
- Higher Quality Content
- 60% Fewer Hallucinations
Browse by: Latest US Patents, China's latest patents, Technical Efficacy Thesaurus, Application Domain, Technology Topic, Popular Technical Reports.
© 2025 PatSnap. All rights reserved.Legal|Privacy policy|Modern Slavery Act Transparency Statement|Sitemap|About US| Contact US: help@patsnap.com