Surface roughness prediction method based on GA-GBRT and method for optimizing process parameters
A surface roughness and prediction method technology, applied in the field of mechanical processing, can solve the problems of reducing surface quality, increasing production costs, unreasonable selection of processing parameters and machine tools, etc., to improve test accuracy, improve processing accuracy, and improve generalization ability Effect
- Summary
- Abstract
- Description
- Claims
- Application Information
AI Technical Summary
Problems solved by technology
Method used
Image
Examples
Embodiment 1
[0116] Example 1. A method for predicting surface roughness based on GA-GBRT is carried out in the following steps:
[0117] a. Collect turning experimental data to form a data set, and divide the data set into training group data and test group data; for example: collect 60 groups of experimental data to form a data set, wherein the training group data is 50 groups, and the test group data is 10 groups;
[0118] Use the training set data to train the key parameters of the GBRT model;
[0119] The data set includes cutting parameters and corresponding surface roughness; the cutting parameters include cutting depth a p , cutting speed V and feed rate f; the key parameters include the number of iterations of promotion M, the maximum depth D of the individual regression estimator and the learning rate v;
[0120] b. Parameter encoding and population initialization: Randomly generate a chromosome sequence for increasing the number of iterations M, the maximum depth D of the indi...
PUM
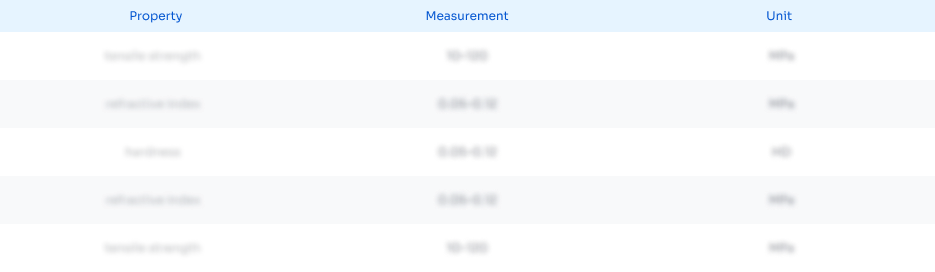
Abstract
Description
Claims
Application Information
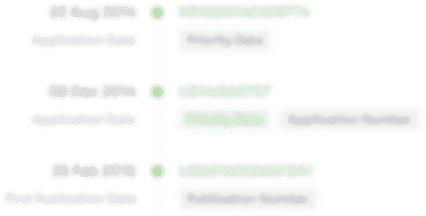
- R&D
- Intellectual Property
- Life Sciences
- Materials
- Tech Scout
- Unparalleled Data Quality
- Higher Quality Content
- 60% Fewer Hallucinations
Browse by: Latest US Patents, China's latest patents, Technical Efficacy Thesaurus, Application Domain, Technology Topic, Popular Technical Reports.
© 2025 PatSnap. All rights reserved.Legal|Privacy policy|Modern Slavery Act Transparency Statement|Sitemap|About US| Contact US: help@patsnap.com