Gaussian process regression-based critical heat flux density prediction method
A technology of critical heat flux and Gaussian process regression, which is applied in the fields of instrumentation, computing, and electrical digital data processing, etc., can solve the problems of falling into the minimum value, difficult structure selection, overfitting and poor promotion ability, etc., and achieve high prediction accuracy Effect
- Summary
- Abstract
- Description
- Claims
- Application Information
AI Technical Summary
Problems solved by technology
Method used
Image
Examples
Embodiment Construction
[0040] The present invention will be described in detail below in conjunction with the accompanying drawings and specific embodiments.
[0041] A kind of critical heat flux prediction method based on Gaussian process regression of the present invention, such as figure 1 As shown, the specific steps are as follows:
[0042] Step 1: Divide the collected data set into two parts, of which 70% of the data is used as the training set, recorded as: where x i ∈R d ,y i ∈R,x i The ith input vector in D includes system pressure P, mass flow rate G and equilibrium steam content Xe, y i Indicates that the i-th target output in D is the critical heat flux; the remaining 30% of the data is used as a test set, denoted as: D * ={(X * ,y * )};
[0043] Step 2: Use the standard normal variable method to preprocess the training set data and test set data, so that the training set D and test set D * The mean value of is 0 and the standard deviation is 1. The calculation formula of prep...
PUM
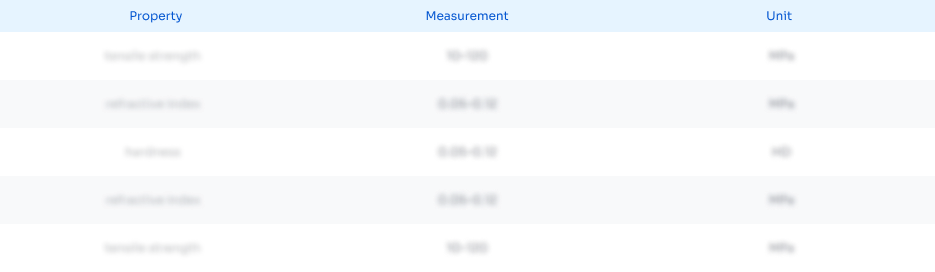
Abstract
Description
Claims
Application Information
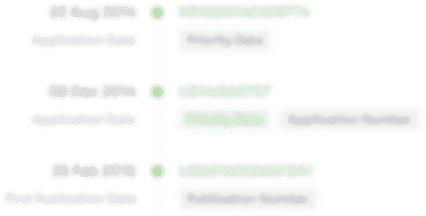
- R&D
- Intellectual Property
- Life Sciences
- Materials
- Tech Scout
- Unparalleled Data Quality
- Higher Quality Content
- 60% Fewer Hallucinations
Browse by: Latest US Patents, China's latest patents, Technical Efficacy Thesaurus, Application Domain, Technology Topic, Popular Technical Reports.
© 2025 PatSnap. All rights reserved.Legal|Privacy policy|Modern Slavery Act Transparency Statement|Sitemap|About US| Contact US: help@patsnap.com