Convolutional Neural Network Hardware Accelerator System Based on Convolution Kernel Splitting and Its Computing Method
A technology of convolutional neural network and hardware accelerator, which is applied in the computing field of large-scale neural network, can solve problems such as inflexibility, achieve the effect of simplifying convolution calculation, reducing the use of computing hardware resources, and avoiding large-scale calculation
- Summary
- Abstract
- Description
- Claims
- Application Information
AI Technical Summary
Problems solved by technology
Method used
Image
Examples
Embodiment Construction
[0034] In this example, if figure 1 As shown, it is a convolutional neural network hardware accelerator system based on the convolution kernel splitting method, which is used to cooperate with the host computer to accelerate large-scale convolution operations in the convolutional neural network through hardware circuits, including: zero padding module, Control module, convolution kernel and data splitting module, convolution kernel weight cache module, data cache module, on-chip address index module, core computing module and intermediate result cache module;
[0035] First, the host will need neural network parameters for accelerated operations, including the number of convolutional layers and pooling layers, the size of input data for each layer, step size, convolution kernel size, weight data and image data stored in off-chip memory The start address of the area is given to the control module. The control module controls the on-chip address index module to generate the add...
PUM
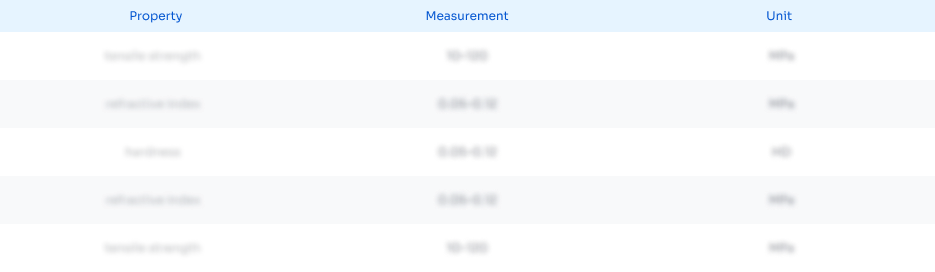
Abstract
Description
Claims
Application Information
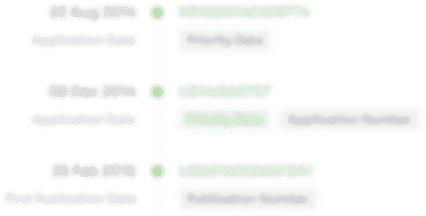
- Generate Ideas
- Intellectual Property
- Life Sciences
- Materials
- Tech Scout
- Unparalleled Data Quality
- Higher Quality Content
- 60% Fewer Hallucinations
Browse by: Latest US Patents, China's latest patents, Technical Efficacy Thesaurus, Application Domain, Technology Topic, Popular Technical Reports.
© 2025 PatSnap. All rights reserved.Legal|Privacy policy|Modern Slavery Act Transparency Statement|Sitemap|About US| Contact US: help@patsnap.com