Prediction method for road network traffic flow based on hierarchical sequence diagram convolutional network
A convolutional network and prediction method technology, applied in the field of deep learning, can solve the problem that the hierarchical structure of spatial factors is not considered, and achieve the effect of improving travel experience, small error, and reducing travel time
- Summary
- Abstract
- Description
- Claims
- Application Information
AI Technical Summary
Problems solved by technology
Method used
Image
Examples
Embodiment Construction
[0048] The following will clearly and completely describe the technical solutions in the embodiments of the present invention with reference to the accompanying drawings in the embodiments of the present invention. Obviously, the described embodiments are only some, not all, embodiments of the present invention. Based on the embodiments of the present invention, all other embodiments obtained by persons of ordinary skill in the art without making creative efforts belong to the protection scope of the present invention.
[0049] Embodiments of the present invention provide a road network traffic flow prediction method based on a hierarchical sequential graph convolutional network, which can greatly improve calculation efficiency on the premise of ensuring the accuracy of traffic flow prediction. The embodiments will be described in detail below in conjunction with the accompanying drawings.
[0050] Such as Figure 1 to Figure 7 As shown, a road network traffic flow prediction...
PUM
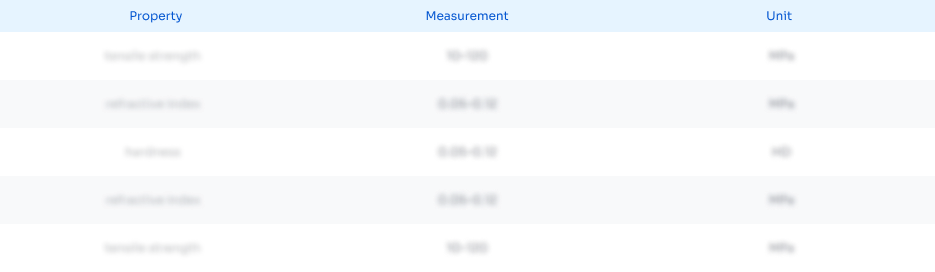
Abstract
Description
Claims
Application Information
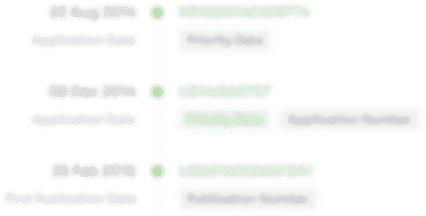
- R&D Engineer
- R&D Manager
- IP Professional
- Industry Leading Data Capabilities
- Powerful AI technology
- Patent DNA Extraction
Browse by: Latest US Patents, China's latest patents, Technical Efficacy Thesaurus, Application Domain, Technology Topic, Popular Technical Reports.
© 2024 PatSnap. All rights reserved.Legal|Privacy policy|Modern Slavery Act Transparency Statement|Sitemap|About US| Contact US: help@patsnap.com