A multivariable time sequence prediction method based on adaptive noise reduction and integrated LSTM
A time series and forecasting method technology, applied in the field of multivariate time series forecasting, can solve the problems of unstable performance of multivariate time series and low forecasting accuracy, achieve high forecasting accuracy and generalization ability, improve forecasting accuracy, avoid The effect of risk of overfitting
- Summary
- Abstract
- Description
- Claims
- Application Information
AI Technical Summary
Problems solved by technology
Method used
Image
Examples
Embodiment Construction
[0019] In order to make the technical solution and advantages of the present invention clearer, further detailed description will be given below in conjunction with the accompanying drawings, but the implementation and protection of the present invention are not limited thereto.
[0020] The multivariate time series prediction method based on adaptive noise reduction and integrated LSTM is divided into three stages: noise reduction stage, feature extraction stage and integrated prediction stage. The flowchart is as follows: figure 1 shown. The specific implementation of each stage will be described in detail below.
[0021] 1. Noise reduction stage
[0022] figure 2 A schematic diagram of the flow chart of adaptive time series noise reduction is shown, and the steps are as follows:
[0023] 1) Target time series decomposition
[0024] Using the Complete Ensemble Empirical Mode Decomposition Method with Adaptive Noise (CEEMDAN) to decompose the target time series, a series...
PUM
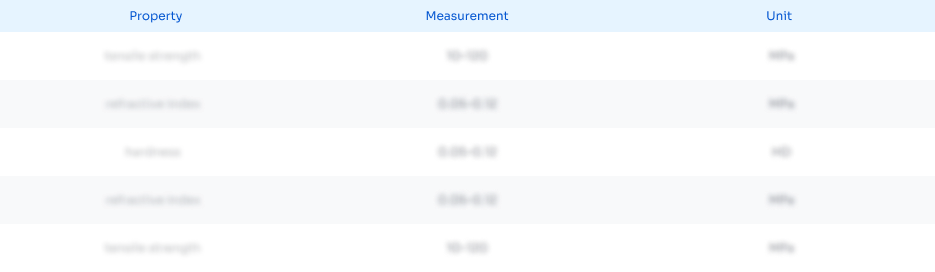
Abstract
Description
Claims
Application Information
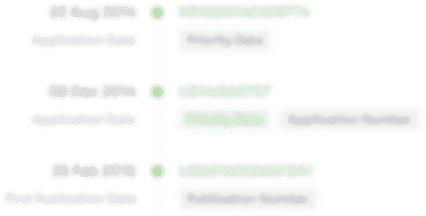
- R&D Engineer
- R&D Manager
- IP Professional
- Industry Leading Data Capabilities
- Powerful AI technology
- Patent DNA Extraction
Browse by: Latest US Patents, China's latest patents, Technical Efficacy Thesaurus, Application Domain, Technology Topic.
© 2024 PatSnap. All rights reserved.Legal|Privacy policy|Modern Slavery Act Transparency Statement|Sitemap