Liver tumor segmentation method and device based on deep learning
A deep learning technology for liver tumors, applied in image analysis, image data processing, instruments, etc., can solve the problems of not considering the spatial structure information of three-dimensional data tumors, mining, and inaccurate segmentation results, and achieve high tumor segmentation accuracy and improve Robustness, the effect of improving segmentation accuracy
- Summary
- Abstract
- Description
- Claims
- Application Information
AI Technical Summary
Problems solved by technology
Method used
Image
Examples
Embodiment Construction
[0025] In order to make the purpose, technical solutions and advantages of the embodiments of the present invention clearer, the technical solutions in the embodiments of the present invention will be clearly and completely described below in conjunction with the drawings in the embodiments of the present invention. Obviously, the described embodiments It is a part of embodiments of the present invention, but not all embodiments. Based on the embodiments of the present invention, all other embodiments obtained by persons of ordinary skill in the art without creative efforts fall within the protection scope of the present invention.
[0026] The embodiment of the present invention combines the fully convolutional neural network and generative adversarial network technology in deep learning, and cooperates with the liver gold standard and tumor gold standard manually drawn by the doctor as the input of the network for learning, so that the network can automatically capture the li...
PUM
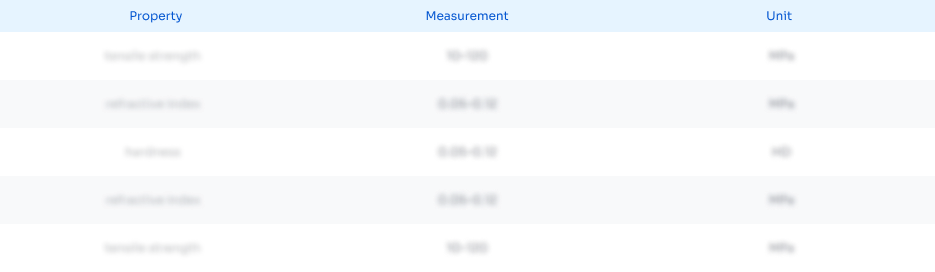
Abstract
Description
Claims
Application Information
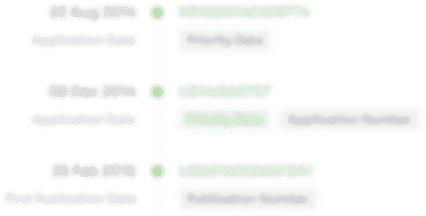
- R&D Engineer
- R&D Manager
- IP Professional
- Industry Leading Data Capabilities
- Powerful AI technology
- Patent DNA Extraction
Browse by: Latest US Patents, China's latest patents, Technical Efficacy Thesaurus, Application Domain, Technology Topic, Popular Technical Reports.
© 2024 PatSnap. All rights reserved.Legal|Privacy policy|Modern Slavery Act Transparency Statement|Sitemap|About US| Contact US: help@patsnap.com