A multi-agent cross-modal depth deterministic strategy gradient training method based on image input
A multi-agent, image input technology, applied in the field of reinforcement learning algorithms, can solve problems such as weak gradient guidance, reduced efficiency of actor network exploration, and impact of training actor exploration efficiency.
- Summary
- Abstract
- Description
- Claims
- Application Information
AI Technical Summary
Problems solved by technology
Method used
Image
Examples
Embodiment 1
[0057] Such as figure 1 As shown, a multi-agent cross-modal depth deterministic policy gradient training method based on image input includes the following steps:
[0058] Step 1. Build the experimental platform in the simulator, define the types of interactive objects and manipulators, define the ultimate goal and reward and punishment rules of the manipulator control task, and clarify the state space and action space of the dual agents;
[0059] Its specific steps include:
[0060] S11. Use the open source simulation platform V-REP to build the experimental environment. The physics engine used is the Vortex open source physics engine. The type of robotic arm used is a UR5 robot with 6 joints;
[0061] S12. Set the task to be completed by the robotic arm control as the grasping task. The task is described as having multiple irregular objects of different sizes, shapes, and colors on the horizontal plane at the same height of the robotic arm. The agent needs to control the ro...
PUM
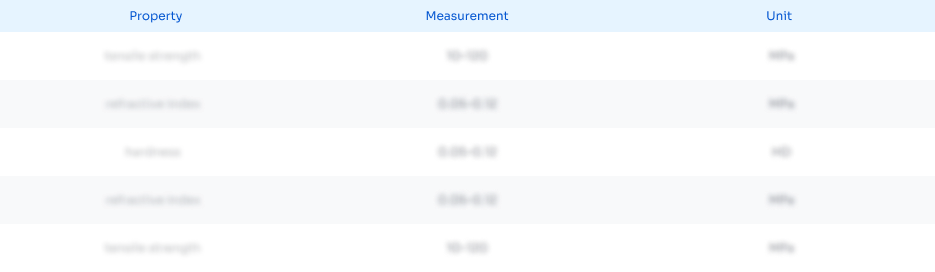
Abstract
Description
Claims
Application Information
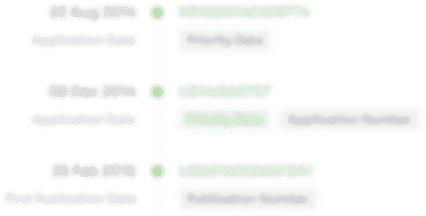
- R&D Engineer
- R&D Manager
- IP Professional
- Industry Leading Data Capabilities
- Powerful AI technology
- Patent DNA Extraction
Browse by: Latest US Patents, China's latest patents, Technical Efficacy Thesaurus, Application Domain, Technology Topic, Popular Technical Reports.
© 2024 PatSnap. All rights reserved.Legal|Privacy policy|Modern Slavery Act Transparency Statement|Sitemap|About US| Contact US: help@patsnap.com