A Deep Learning Accelerator for Stacked Hourglass Networks
A deep learning and stacking technology, applied in the field of neural network training, can solve the problems of occupying hardware running time, reducing battery life, and not being able to accelerate, so as to improve efficiency, improve utilization, and reduce delay.
- Summary
- Abstract
- Description
- Claims
- Application Information
AI Technical Summary
Problems solved by technology
Method used
Image
Examples
Embodiment Construction
[0046] The preferred embodiments of the present invention will be described below in conjunction with the accompanying drawings. It should be understood that the preferred embodiments described here are only used to illustrate and explain the present invention, and are not intended to limit the present invention.
[0047] Such as figure 1 As shown, this embodiment discloses a deep learning accelerator suitable for a stacked hourglass network, including a control module 1, a data calculation module 2 and a data cache module 3;
[0048] The control module 1 is connected to the main control processor, and is used to receive the control signal input by the main control processor, and control the data calculation module 2 and the data cache module 3 according to the control signal;
[0049] Specifically, such as figure 2 As shown, the data calculation module 2 includes a plurality of layer calculation units 21; the layer calculation units 21 are used to perform data processing op...
PUM
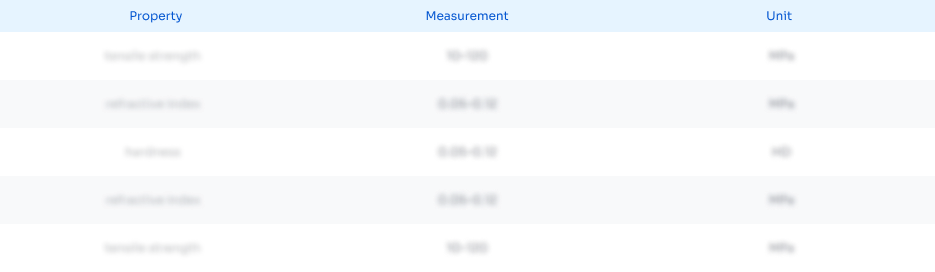
Abstract
Description
Claims
Application Information
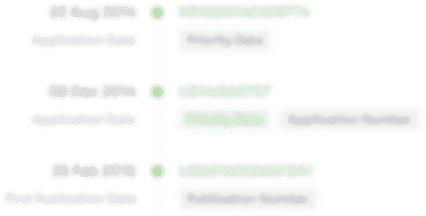
- R&D Engineer
- R&D Manager
- IP Professional
- Industry Leading Data Capabilities
- Powerful AI technology
- Patent DNA Extraction
Browse by: Latest US Patents, China's latest patents, Technical Efficacy Thesaurus, Application Domain, Technology Topic, Popular Technical Reports.
© 2024 PatSnap. All rights reserved.Legal|Privacy policy|Modern Slavery Act Transparency Statement|Sitemap|About US| Contact US: help@patsnap.com