Monocular image depth-of-field real-time calculation method based on unsupervised deep learning
A deep learning and real-time computing technology, applied in the field of artificial intelligence, can solve the problems such as the difficulty of obtaining a large number of artificially labeled data sets and the limited application scenarios, and achieve the effect of improving the accuracy and robustness, and improving the accuracy of the algorithm.
- Summary
- Abstract
- Description
- Claims
- Application Information
AI Technical Summary
Problems solved by technology
Method used
Image
Examples
Embodiment Construction
[0030] In order to make the object, technical solution and advantages of the present invention clearer, the present invention will be further described in detail below in combination with specific examples and with reference to the accompanying drawings.
[0031] A real-time calculation method of monocular image depth of field based on unsupervised deep learning, such as figure 1 As shown, it specifically includes the following steps:
[0032] Step 1: Training data preprocessing.
[0033] Using the binocular sequence images in the unmanned driving dataset KITTI as input data, the required images are classified into two types through data preprocessing: (1) stereo image pairs for Depth-CNN network; (2) for Sequence images of Pose-CNN network.
[0034]To preprocess all the data in the KITTI database, first convert the original image into an image with a size of 256×512, and the gray value of the image on the three channels of R, G, and B is between 0 and 1. Reorganize data ac...
PUM
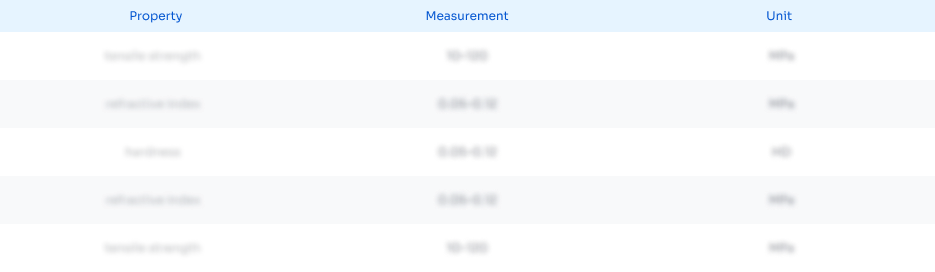
Abstract
Description
Claims
Application Information
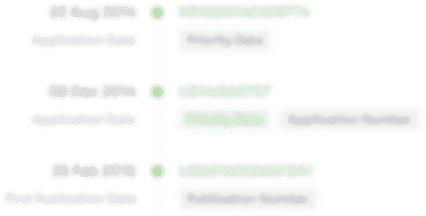
- R&D Engineer
- R&D Manager
- IP Professional
- Industry Leading Data Capabilities
- Powerful AI technology
- Patent DNA Extraction
Browse by: Latest US Patents, China's latest patents, Technical Efficacy Thesaurus, Application Domain, Technology Topic.
© 2024 PatSnap. All rights reserved.Legal|Privacy policy|Modern Slavery Act Transparency Statement|Sitemap