Robustness sorting learning method based on multi-objective particle swarm optimization and application thereof
A multi-objective particle swarm and ranking learning technology, which is applied in the fields of information retrieval and machine learning, can solve problems such as unstable sorting systems, poor performance, and unstable sorting results
- Summary
- Abstract
- Description
- Claims
- Application Information
AI Technical Summary
Problems solved by technology
Method used
Image
Examples
specific Embodiment 1
[0178] Such as Figure 5 As shown, it is assumed that there is a standard sorting learning dataset L2Rdataset, expressed as a set: L2Rdataset={(i , d ij >,y ij )|q i ∈Q,d ij ∈D i ,y ij ∈ Y i ,1≤i≤|Q|,1≤j≤|D i |}, where q i Represents the i-th query, Q represents the limited query set in the ranking learning dataset, |Q| represents the total number of queries in the query set, d ij Represents a collection of documents D i The jth document in D, D i Indicates that it is associated with the query q i collection of documents, |D i |Represents a collection of documents D i The total number of documents in y ij Represents a relevance annotation, reflecting the query q i and document d ij The degree of correlation between, the value can be some level value, such as {1, 2, 3, 4, 5}, Y i ={y i1 ,y i2 ,...,y i|Di|} represents the tag set associated with the query. query-document pair i , d ij > Described by the M-dimensional sorting feature f, which can be expressed...
specific Embodiment 2
[0180] Such as Figure 6 As shown, a robust ranking learning method based on multi-objective particle swarm optimization can be applied to the actual demand sorting application scenarios such as information retrieval, search engine, recommendation system and question answering system. Here, the robust ranking learning method based on multi-objective particle swarm optimization is applied to search engines such as Baidu, Google, Bing, Sogou and Yahoo, as an application example. The ranking model trained by this method is embedded in the ranking system of the search engine, and the ranking model is used to predict the ranking results of the web pages of the query words that users need to search, so as to improve the overall user satisfaction and enhance the user experience.
[0181] The implementation process of the robust ranking learning method based on multi-objective particle swarm optimization applied to the search engine is as follows: Figure 6 As shown, its implementat...
PUM
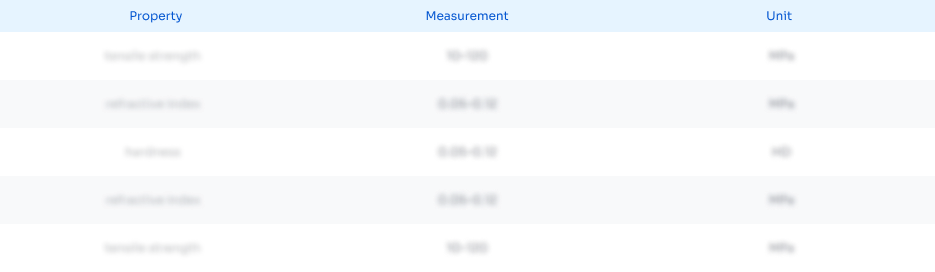
Abstract
Description
Claims
Application Information
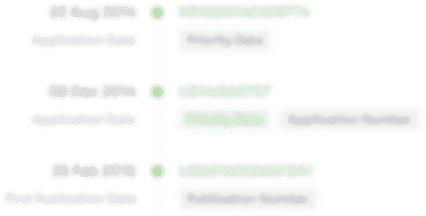
- R&D
- Intellectual Property
- Life Sciences
- Materials
- Tech Scout
- Unparalleled Data Quality
- Higher Quality Content
- 60% Fewer Hallucinations
Browse by: Latest US Patents, China's latest patents, Technical Efficacy Thesaurus, Application Domain, Technology Topic, Popular Technical Reports.
© 2025 PatSnap. All rights reserved.Legal|Privacy policy|Modern Slavery Act Transparency Statement|Sitemap|About US| Contact US: help@patsnap.com