Electroencephalogram signal rapid identification method of dense deep convolutional neural network
A convolutional neural network and neural network technology, applied in the field of signal processing and pattern recognition, can solve the problems of inability to extract deeper features, overfitting, low model accuracy, etc., to solve the problem of gradient disappearance, support feature reuse, The effect of avoiding information loss
- Summary
- Abstract
- Description
- Claims
- Application Information
AI Technical Summary
Problems solved by technology
Method used
Image
Examples
Embodiment Construction
[0036] The present invention will be further described in detail below in conjunction with the accompanying drawings and specific embodiments. The present invention mainly utilizes the weight sharing of the convolutional neural network and the local receptive field idea, and connects the channels of the output feature map of the middle layer to improve the recognition accuracy. Each neuron of the convolutional neural network uses the same convolution kernel when convoluting different feature maps, which will greatly reduce the amount of weight parameters. After the convolution operation, the input and output of the convolution are connected to realize the feature. Reuse, so that only very few new feature maps are generated after convolution, so as to reduce redundancy. The dense deep convolutional neural network of the present invention uses the stochastic gradient descent method to backpropagate errors, adjusts the weight of the convolution kernel, and finally obtains the pro...
PUM
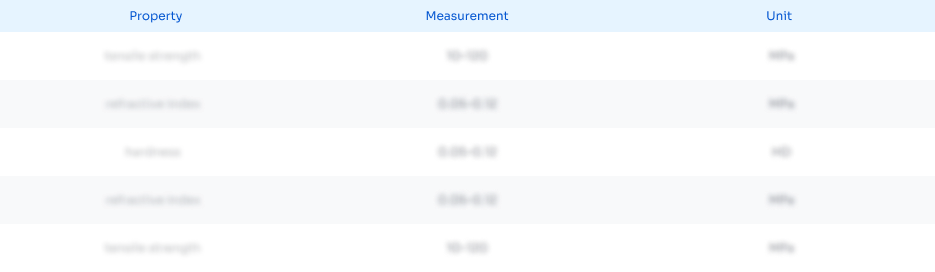
Abstract
Description
Claims
Application Information
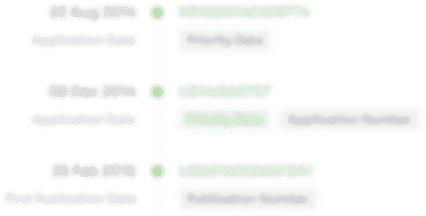
- R&D Engineer
- R&D Manager
- IP Professional
- Industry Leading Data Capabilities
- Powerful AI technology
- Patent DNA Extraction
Browse by: Latest US Patents, China's latest patents, Technical Efficacy Thesaurus, Application Domain, Technology Topic, Popular Technical Reports.
© 2024 PatSnap. All rights reserved.Legal|Privacy policy|Modern Slavery Act Transparency Statement|Sitemap|About US| Contact US: help@patsnap.com