Power load prediction method based on LSTM and LGBM
A technology of power load and forecasting method, which is applied in the field of power load forecasting based on LSTM and LGBM, can solve problems such as the generalization of unfavorable methods, and achieve the effects of improving forecasting accuracy, good timing memory ability, and training stability
- Summary
- Abstract
- Description
- Claims
- Application Information
AI Technical Summary
Problems solved by technology
Method used
Image
Examples
Embodiment Construction
[0044] In order to make the technical solutions of the present invention clearer and clearer to those skilled in the art, the present invention will be further described in detail below in conjunction with the examples and accompanying drawings, but the embodiments of the present invention are not limited thereto.
[0045] Such as Figure 1-Figure 7 As shown, the power load forecasting method based on LSTM and LGBM provided in this embodiment proposes a power forecasting model based on Long and Short Term Memory (LSTM), and optimizes the parameters in combination with the error backpropagation rule To solve the problem, build a power load forecasting model based on the decision tree gradient boosting method LGBM to reduce the shortcomings of a single forecasting model in terms of forecasting performance, and at the same time learn from the idea of boosting ensemble learning to further improve forecasting accuracy.
[0046] The models in this example are all implemented using...
PUM
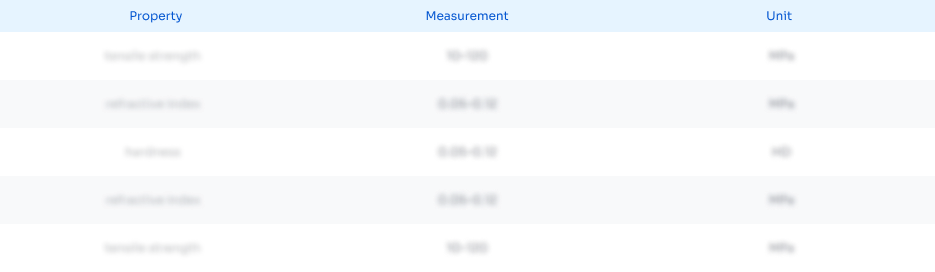
Abstract
Description
Claims
Application Information
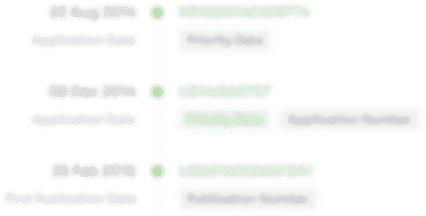
- R&D Engineer
- R&D Manager
- IP Professional
- Industry Leading Data Capabilities
- Powerful AI technology
- Patent DNA Extraction
Browse by: Latest US Patents, China's latest patents, Technical Efficacy Thesaurus, Application Domain, Technology Topic.
© 2024 PatSnap. All rights reserved.Legal|Privacy policy|Modern Slavery Act Transparency Statement|Sitemap