Asynchronous motor fault monitoring and diagnosing method based on deep learning
An asynchronous motor and fault monitoring technology, applied in neural learning methods, motor generator testing, biological neural network models, etc., can solve problems such as cumbersome steps, and achieve the effect of lowering the threshold, high fault diagnosis accuracy, and saving development time.
- Summary
- Abstract
- Description
- Claims
- Application Information
AI Technical Summary
Problems solved by technology
Method used
Image
Examples
Embodiment Construction
[0051]The following is a detailed description of the embodiments of the present invention. This embodiment is carried out based on the technical solution of the present invention, and provides detailed implementation methods and specific operation processes to further explain the technical solution of the present invention.
[0052] The invention provides a deep learning-based asynchronous motor fault monitoring and diagnosis method, including two processes of LRCN-LSTM deep neural network model establishment and real-time operating state monitoring.
[0053] Wherein, described deep neural network establishment process comprises the following steps:
[0054] Step S1, data preprocessing;
[0055] Step S1.1, raw data sampling;
[0056] The sampling frequency of the original power load data used in the present invention is 20kHz, and the rated operating frequency of alternating current 50Hz is selected as the fundamental frequency of the power load time series (that is, each pow...
PUM
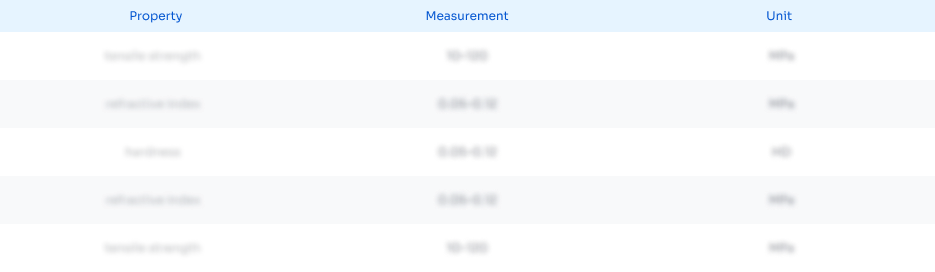
Abstract
Description
Claims
Application Information
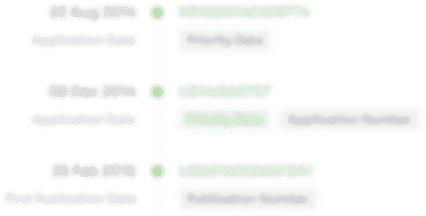
- R&D
- Intellectual Property
- Life Sciences
- Materials
- Tech Scout
- Unparalleled Data Quality
- Higher Quality Content
- 60% Fewer Hallucinations
Browse by: Latest US Patents, China's latest patents, Technical Efficacy Thesaurus, Application Domain, Technology Topic, Popular Technical Reports.
© 2025 PatSnap. All rights reserved.Legal|Privacy policy|Modern Slavery Act Transparency Statement|Sitemap|About US| Contact US: help@patsnap.com