Noise diagnosis algorithm for rolling bearing faults of rotary equipment
A technology of rolling bearings and rotating equipment, which is applied in computing, computer components, measuring devices, etc., and can solve problems such as economic losses of enterprises, environmental hazards, and endangering the lives of employees.
- Summary
- Abstract
- Description
- Claims
- Application Information
AI Technical Summary
Problems solved by technology
Method used
Image
Examples
Embodiment
[0060] The noise data of the rotating equipment under normal operation was collected through the network pickup, and according to the prior knowledge, based on the Gaussian probability model, the Gibbs sampling was used to generate some fault test samples such as the inner ring, the ball, the outer ring, etc., to solve the problem of fault samples in actual operation. less impact on learning outcomes. The motor speed is 1800rpm, and the noise sampling frequency is 44100Hz. The measured signal is divided into one frame every 256 points, and 1500 frames are intercepted for each type of signal, with a total of 6000 frames of data. The MFCC features are extracted for each frame, and the feature dimension is set to 12, forming 6000 samples. Divide 6000 samples into training samples and test samples, in which the number of training samples is 4000 (each class has 1000 samples), and the number of test samples is 2000 (each class has 500 samples). in, figure 2 Four-dimensional eig...
PUM
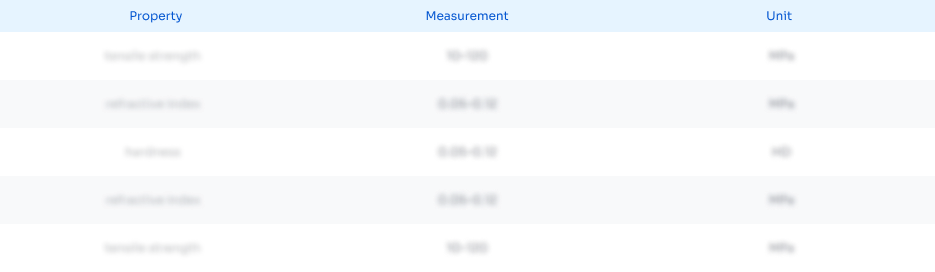
Abstract
Description
Claims
Application Information
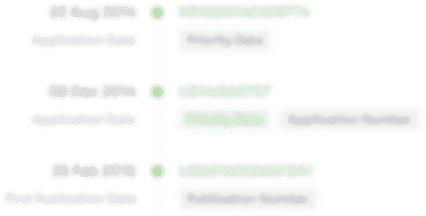
- R&D Engineer
- R&D Manager
- IP Professional
- Industry Leading Data Capabilities
- Powerful AI technology
- Patent DNA Extraction
Browse by: Latest US Patents, China's latest patents, Technical Efficacy Thesaurus, Application Domain, Technology Topic, Popular Technical Reports.
© 2024 PatSnap. All rights reserved.Legal|Privacy policy|Modern Slavery Act Transparency Statement|Sitemap|About US| Contact US: help@patsnap.com