Data noise suppressing method based on residual block full convolutional neural network
A convolutional neural network and noise suppression technology, applied to biological neural network models, neural architectures, measurement devices, etc., can solve the problems of seismic data generalization limitations, model generalization limitations, etc., to achieve sufficient learning and generalization obvious effect
- Summary
- Abstract
- Description
- Claims
- Application Information
AI Technical Summary
Problems solved by technology
Method used
Image
Examples
Embodiment Construction
[0019] In order to effectively remove random noise in seismic data, this paper proposes a RUnet convolutional neural network denoising model, including the following steps.
[0020] Step 1: The noise-containing seismic data with different noise levels and the preprocessed 3D post-stack seismic data are used as the training set. The specific steps are as follows:
[0021] (1) Select 256 traces from the Parihaka post-stack 3D seismic data volume, and the sampling points are 256 seismic data slices;
[0022] (2) Add 20%, 25%, and 30% Gaussian random noise to the seismic data respectively, and use the corresponding preprocessed seismic data as a training set, where the noise-added seismic data is used as input, and the preprocessed seismic data is used as label, the sample size is 900;
[0023] Step 2: The network as a whole includes an encoding process and a decoding process. The encoding process consists of 5 groups of residual blocks, each group of residual blocks consists of...
PUM
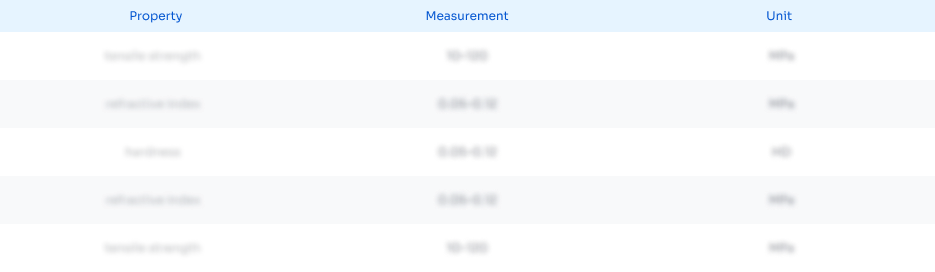
Abstract
Description
Claims
Application Information
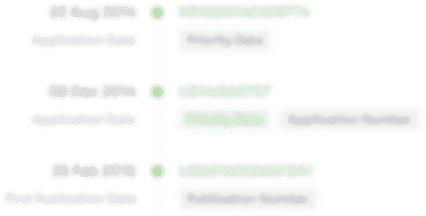
- R&D
- Intellectual Property
- Life Sciences
- Materials
- Tech Scout
- Unparalleled Data Quality
- Higher Quality Content
- 60% Fewer Hallucinations
Browse by: Latest US Patents, China's latest patents, Technical Efficacy Thesaurus, Application Domain, Technology Topic, Popular Technical Reports.
© 2025 PatSnap. All rights reserved.Legal|Privacy policy|Modern Slavery Act Transparency Statement|Sitemap|About US| Contact US: help@patsnap.com