Cellular network traffic prediction method based on three-dimensional convolutional neural network
A neural network and three-dimensional convolution technology, applied in neural learning methods, biological neural network models, neural architectures, etc., can solve the problem of inability to model nonlinear relationships, ignore potential correlations of traffic sequences, and fail to capture rapid changes in underlying traffic loads. It can improve the training time and prediction accuracy, and reduce the network parameters.
- Summary
- Abstract
- Description
- Claims
- Application Information
AI Technical Summary
Problems solved by technology
Method used
Image
Examples
Embodiment Construction
[0044] The following will clearly and completely describe the technical solutions in the embodiments of the present invention with reference to the accompanying drawings in the embodiments of the present invention. Obviously, the described embodiments are only some, not all, embodiments of the present invention. Based on the embodiments of the present invention, all other embodiments obtained by persons of ordinary skill in the art without making creative efforts belong to the protection scope of the present invention.
[0045] Please refer to Figure 1 to Figure 4 , the present invention provides a method for predicting traffic in a cellular network based on a three-dimensional convolutional neural network, comprising the following steps:
[0046] S1: Modeling network traffic data as a three-dimensional tensor input form to obtain a three-dimensional network traffic data model, the three-dimensional network traffic data model includes long-term dependent data and short-term d...
PUM
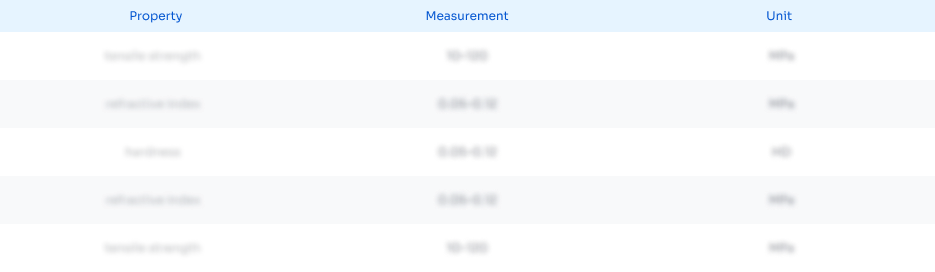
Abstract
Description
Claims
Application Information
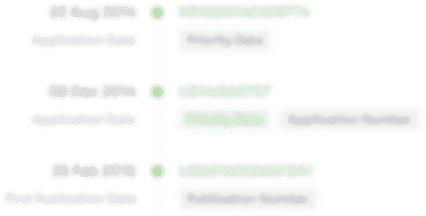
- R&D Engineer
- R&D Manager
- IP Professional
- Industry Leading Data Capabilities
- Powerful AI technology
- Patent DNA Extraction
Browse by: Latest US Patents, China's latest patents, Technical Efficacy Thesaurus, Application Domain, Technology Topic, Popular Technical Reports.
© 2024 PatSnap. All rights reserved.Legal|Privacy policy|Modern Slavery Act Transparency Statement|Sitemap|About US| Contact US: help@patsnap.com