Dynamic missing value filling method based on detracking auto-encoder
An autoencoder and missing value technology, applied in neural learning methods, neural architectures, biological neural network models, etc., can solve problems such as reduced training accuracy, achieve structural simplicity, improve known information utilization, and improve regression performance effect
- Summary
- Abstract
- Description
- Claims
- Application Information
AI Technical Summary
Problems solved by technology
Method used
Image
Examples
Embodiment Construction
[0043] The specific embodiments of the present invention will be described in detail below in combination with the summary of the invention and the accompanying drawings.
[0044] figure 1 It is a working flow diagram of the present invention. In the figure, the first row A in the incomplete data set 1 ,A 2 ,A 3 ,...,A s Indicates attribute names, and black markers indicate missing values. based on figure 1 It can be seen that the present invention builds the network model TRAE according to the number of attributes of the data set, and then uses the filling scheme MVPT to realize network training and filling of missing values in parallel. Before training, the scheme randomly initializes the network parameters and missing value estimates; during the training process, the entire incomplete data set is input into TRAE as a training set; TRAE updates network parameters and missing value estimates based on the optimization algorithm; the updated missing value estimates are ...
PUM
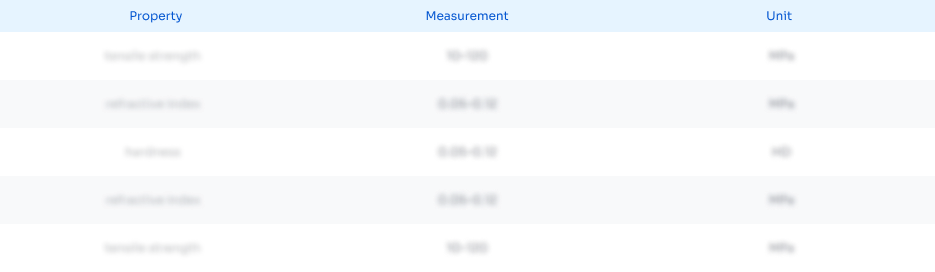
Abstract
Description
Claims
Application Information
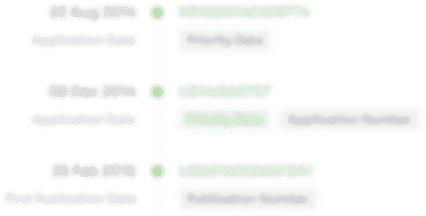
- R&D
- Intellectual Property
- Life Sciences
- Materials
- Tech Scout
- Unparalleled Data Quality
- Higher Quality Content
- 60% Fewer Hallucinations
Browse by: Latest US Patents, China's latest patents, Technical Efficacy Thesaurus, Application Domain, Technology Topic, Popular Technical Reports.
© 2025 PatSnap. All rights reserved.Legal|Privacy policy|Modern Slavery Act Transparency Statement|Sitemap|About US| Contact US: help@patsnap.com