Faster-RCNN (Recurrent Convolutional Neural Network) and Kalman filtering combined moving human body tracking method
A Kalman filter, human body technology, applied in the field of image processing, can solve the problems of tracking failure, affecting the real-time tracking of moving human bodies, etc., to achieve the effect of improving real-time performance, high tracking accuracy, and strong robustness
- Summary
- Abstract
- Description
- Claims
- Application Information
AI Technical Summary
Problems solved by technology
Method used
Image
Examples
Embodiment
[0059] (1) Simplification of Faster-RCNN network
[0060] The original Faster-RCNN network generally includes three parts: the convolutional neural network, the RPN network and the fully connected layer. Since the fully connected layer contains millions of parameters when performing feature classification, the running speed is slow, and it does not meet the real-time requirements of moving human body tracking in a dynamic background. Therefore, it is necessary to remove the fully connected layer when simplifying the Faster-RCNN network. part.
[0061] 1.1) Acquisition of convolutional feature maps
[0062] Before the input image enters the convolutional neural network, in order to ensure the consistency of the output vector, it needs to be resized, and all input images are resized to 800×600. Then send the picture to the trained convolutional neural network (VGG16) to get a complete convolutional feature map of the input image.
[0063] 1.2) Acquisition of moving human body...
Embodiment approach
[0099] The embodiment of the present invention comprises the following steps:
[0100] (1) Simplification of Faster-RCNN network
[0101] The original Faster-RCNN network generally includes three parts: the convolutional neural network, the RPN network and the fully connected layer. Since the fully connected layer contains millions of parameters when performing feature classification, the running speed is slow, and it does not meet the real-time requirements of moving human body tracking in a dynamic background. Therefore, it is necessary to remove the fully connected layer when simplifying the Faster-RCNN network. part.
[0102] 1.1) Acquisition of convolutional feature maps
[0103] Before the input image enters the convolutional neural network, in order to ensure the consistency of the output vector, it needs to be resized, and all input images are resized to 800×600. Then send the picture to the trained convolutional neural network (VGG16) to get a complete convolutiona...
PUM
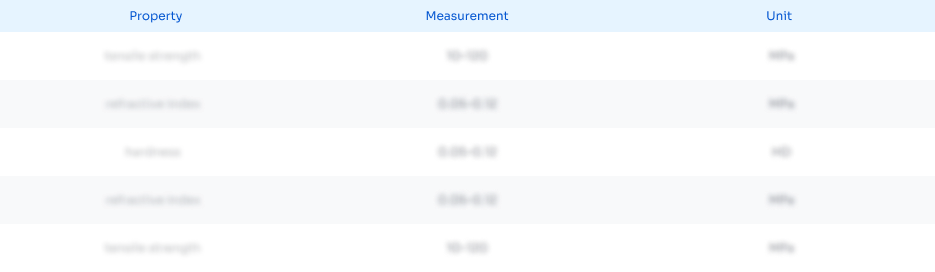
Abstract
Description
Claims
Application Information
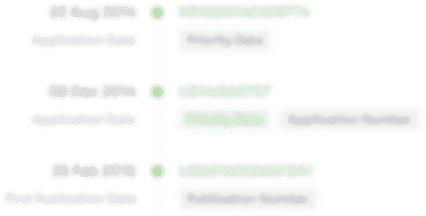
- R&D Engineer
- R&D Manager
- IP Professional
- Industry Leading Data Capabilities
- Powerful AI technology
- Patent DNA Extraction
Browse by: Latest US Patents, China's latest patents, Technical Efficacy Thesaurus, Application Domain, Technology Topic, Popular Technical Reports.
© 2024 PatSnap. All rights reserved.Legal|Privacy policy|Modern Slavery Act Transparency Statement|Sitemap|About US| Contact US: help@patsnap.com