Short video personalized recommendation method and system based on multi-modal graph convolutional network
A technology of convolutional network and recommendation method, which is applied in the field of short video personalized recommendation based on multimodal graph convolutional network, can solve the problem of ineffective and poor accuracy of short video personalized recommendation, inability to express multimodal content information, and user Preference expression deviation and other issues to achieve the effect of improving accuracy and comprehensibility, accurate and effective personalized recommendation
- Summary
- Abstract
- Description
- Claims
- Application Information
AI Technical Summary
Problems solved by technology
Method used
Image
Examples
Embodiment 1
[0043] figure 1 The flow chart of the short video personalized recommendation method based on the multi-modal graph convolutional network of this embodiment is given.
[0044] Such as figure 1 As shown, the short video personalized recommendation method based on the multimodal graph convolutional network in this embodiment includes:
[0045]S101: Build a user-short video graph structure based on the image mode, audio mode, and text mode of the short video; the points in the user-short video graph structure represent the user and the short video, and the lines between the points represent both interaction between.
[0046] In the specific implementation, data sets are randomly extracted from the three platforms of Douyin, Kuaishou and Movielens, including users, short videos and users' playback history.
[0047] According to the user's playback history, on each modality m (including image v, audio a and text t), namely Build the graph structure separately Points in the g...
Embodiment 2
[0094] figure 2 A schematic structural diagram of the short video personalized recommendation system based on the multi-modal graph convolutional network of this embodiment is given.
[0095] Such as figure 2 As shown, the short video personalized recommendation system based on the multimodal graph convolutional network of this embodiment includes:
[0096] (1) User-short video graph structure building module, which is used to construct the user-short video graph structure based on the image mode, audio mode and text mode of the short video respectively; the point in the user-short video graph structure represents the user and short videos, the connection between the dots indicates the interaction between the two;
[0097] (2) User and short video expression module, which is used to input the user-short video graph structure of each mode into the corresponding graph convolutional neural network, and calculate and express each modality through the aggregation layer of the c...
Embodiment 3
[0105] This embodiment provides a computer-readable storage medium, on which a computer program is stored, and when the program is executed by a processor, the following figure 1 The steps in the short video personalized recommendation method based on multimodal graph convolutional network are shown.
[0106] In this embodiment, through the construction of a multimodal graph convolutional neural network, the user's preferences in different modalities are modeled, and at the same time, the multimodal content information is used to express the user's preferences. On this basis, different modalities According to the fusion of user preferences, the interest expression of users is obtained, and the relationship between user preferences and short video content information is calculated to provide users with more accurate short video personalized recommendations.
PUM
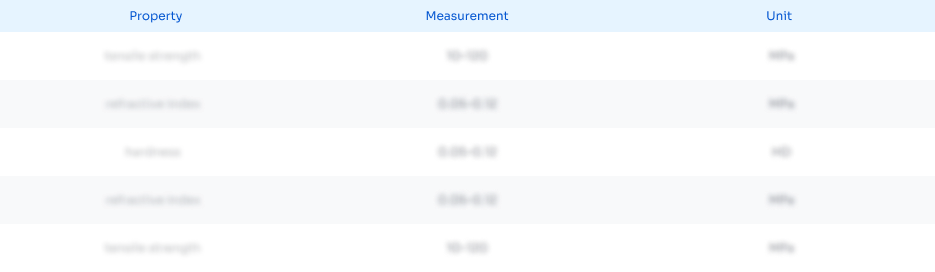
Abstract
Description
Claims
Application Information
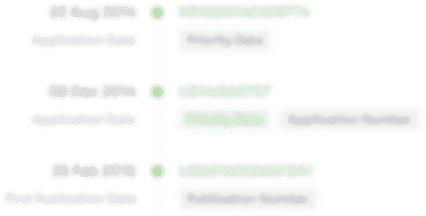
- R&D
- Intellectual Property
- Life Sciences
- Materials
- Tech Scout
- Unparalleled Data Quality
- Higher Quality Content
- 60% Fewer Hallucinations
Browse by: Latest US Patents, China's latest patents, Technical Efficacy Thesaurus, Application Domain, Technology Topic, Popular Technical Reports.
© 2025 PatSnap. All rights reserved.Legal|Privacy policy|Modern Slavery Act Transparency Statement|Sitemap|About US| Contact US: help@patsnap.com