Arhythmia testing method for aiming at electrocardiogram data by means of binary neural network
A binary nerve and arrhythmia technology, applied in the field of deep learning, can solve the problem of high computing cost and achieve the effect of reducing computing memory, low computing power, and low energy consumption
- Summary
- Abstract
- Description
- Claims
- Application Information
AI Technical Summary
Problems solved by technology
Method used
Image
Examples
Embodiment Construction
[0025] Such as figure 1 Shown, the specific content of the present invention is described below.
[0026] Step 1. Obtain the content of the training data. The data set used comes from the atrial fibrillation (AF) recognition competition held by PhysioNet in 2017, which contains 8528 single-lead ECG data, ranging in length from 9 seconds to 61 seconds. The sampling frequency is 300Hz. The data is labeled by experts and divided into four categories: Normal rhythm (N), AF rhythm (A), Other rhythm (O) and Noise (~). The number of samples in the four categories is uneven, of which Normal has 5154 , AF has 771, Other has 2557, and Noise has 46. Examples of different categories of data are as follows image 3 shown. Symptoms of arrhythmia that are not atrial fibrillation are grouped under other arrhythmias. Due to the different lengths of data, it is impossible to meet the requirements of batch training. Therefore, the data is first filled with data in batches. In addition, beca...
PUM
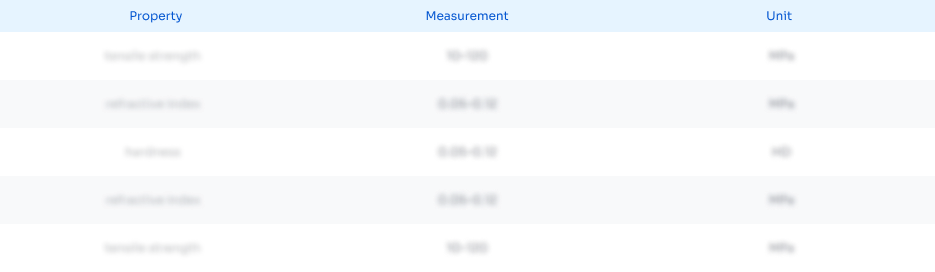
Abstract
Description
Claims
Application Information
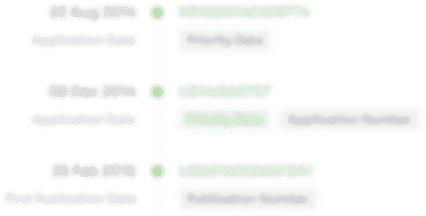
- R&D Engineer
- R&D Manager
- IP Professional
- Industry Leading Data Capabilities
- Powerful AI technology
- Patent DNA Extraction
Browse by: Latest US Patents, China's latest patents, Technical Efficacy Thesaurus, Application Domain, Technology Topic, Popular Technical Reports.
© 2024 PatSnap. All rights reserved.Legal|Privacy policy|Modern Slavery Act Transparency Statement|Sitemap|About US| Contact US: help@patsnap.com