Continuous casting billet surface defect binocular scanning and deep learning fusion recognition method and system
A defect identification and deep learning technology, which is applied in the field of continuous casting slab surface defect binocular scanning and deep learning fusion identification method and system, to achieve the effect of solving three-dimensional shape information distortion, reducing regional difficulty, and three-dimensional quantitative reliable detection.
- Summary
- Abstract
- Description
- Claims
- Application Information
AI Technical Summary
Problems solved by technology
Method used
Image
Examples
Embodiment Construction
[0020] The specific embodiments of the present invention will be described below to further illustrate the starting point and corresponding technical solutions of the present invention.
[0021] figure 1 It is a flow chart of a binocular scanning and deep learning fusion recognition method for surface defects of continuous casting slabs provided by an embodiment of the present invention. The method includes the following steps:
[0022] Step 101, using binocular laser scanning imaging to extract the three-dimensional topography image of the continuous casting slab surface;
[0023] Step 102, for the three-dimensional topography image, locate the region of interest ROI according to the depth information, and generate a defect recognition candidate frame;
[0024] Step 103 , for the defect identification candidate frame, use a fully-connected neural network to perform real defect discrimination and type identification on the defect area in the candidate frame, and use a fully c...
PUM
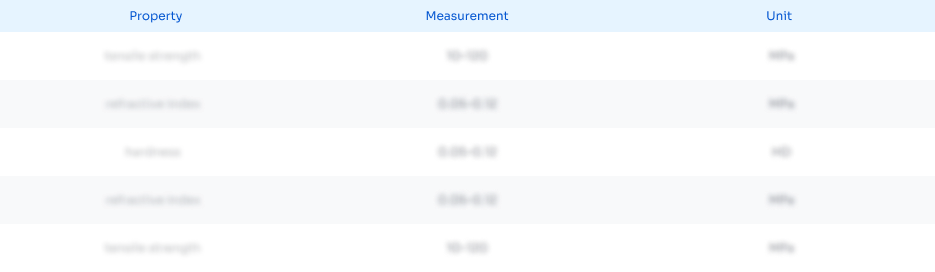
Abstract
Description
Claims
Application Information
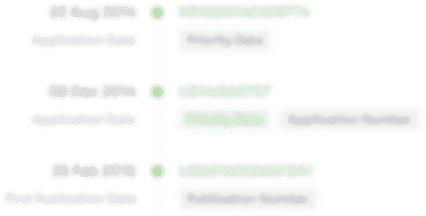
- R&D
- Intellectual Property
- Life Sciences
- Materials
- Tech Scout
- Unparalleled Data Quality
- Higher Quality Content
- 60% Fewer Hallucinations
Browse by: Latest US Patents, China's latest patents, Technical Efficacy Thesaurus, Application Domain, Technology Topic, Popular Technical Reports.
© 2025 PatSnap. All rights reserved.Legal|Privacy policy|Modern Slavery Act Transparency Statement|Sitemap|About US| Contact US: help@patsnap.com