Answer selection model based on internal attention mechanism of GRU neural network
A neural network and model selection technology, applied in the field of algorithms for selecting optimal responses, to achieve the effect of improving the stability of the algorithm
- Summary
- Abstract
- Description
- Claims
- Application Information
AI Technical Summary
Problems solved by technology
Method used
Image
Examples
Embodiment 1
[0026] figure 1 is a schematic structural diagram of a recurrent neural network RNN according to an embodiment of the present invention. Recurrent Neural Networks (RNN) is a common artificial neural network. The connection lines between nodes in the network form a directed ring. RNN has important applications in many natural language processing tasks. Different from the feed-forward neural network (Feed-forward Neural Networks, FNN) in which the input and output are independent of each other, RNN can effectively use the output of the previous moment. Therefore, RNN is more suitable for processing sequence data. In theory, RNNs can handle arbitrarily long sequences, but in practice they cannot. RNN has achieved very good results in tasks such as language model, text generation, machine translation, language recognition and image description generation.
[0027] figure 2 is a schematic structural diagram of a traditional GRU according to an embodiment of the present inven...
Embodiment
[0054] The present invention has carried out precision comparison and analysis experiment to above-mentioned method and traditional method, specifically as follows:
[0055] This experiment uses Chinese data translated from the insuranceQA corpus. Before the experiment, the questions and answers were first cut into words, and then word2vec was used to pre-train the questions and answers. Since this experiment uses a fixed-length GRU, the questions and answers need to be truncated (too long) or supplemented (too short).
[0056] Experimental modeling generates input data. This experiment is modeled in the form of a question-answer triplet (q, a+, a-), where q represents a question, a+ represents a positive answer, and a- represents a negative answer. The training data in insuranceQA already contains questions and positive answers, so it is necessary to select the negative answers. In the experiment, we randomly selected the negative answers and combined them into the form of ...
PUM
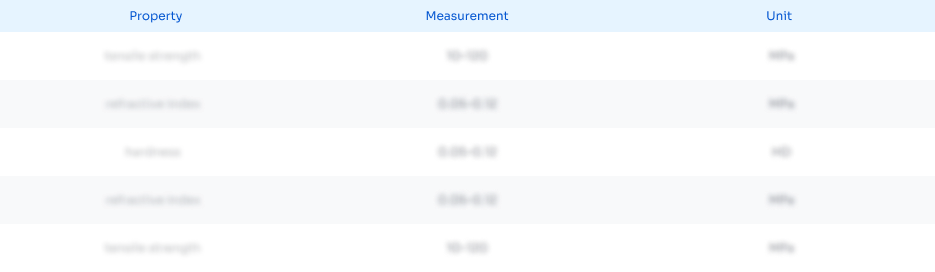
Abstract
Description
Claims
Application Information
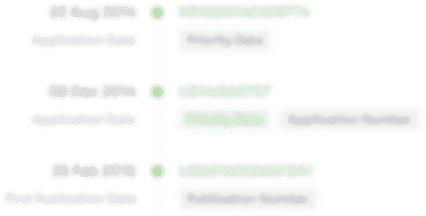
- Generate Ideas
- Intellectual Property
- Life Sciences
- Materials
- Tech Scout
- Unparalleled Data Quality
- Higher Quality Content
- 60% Fewer Hallucinations
Browse by: Latest US Patents, China's latest patents, Technical Efficacy Thesaurus, Application Domain, Technology Topic, Popular Technical Reports.
© 2025 PatSnap. All rights reserved.Legal|Privacy policy|Modern Slavery Act Transparency Statement|Sitemap|About US| Contact US: help@patsnap.com