Method for classifying electroencephalogram (EEG) signals based on multi-scale brain function network
A brain function network and EEG signal technology, applied in the field of EEG signal classification based on multi-scale information fusion
- Summary
- Abstract
- Description
- Claims
- Application Information
AI Technical Summary
Problems solved by technology
Method used
Image
Examples
Embodiment Construction
[0043] The flow chart of the method for classifying EEG signals based on the multi-scale brain function network of the present invention is as follows figure 1 shown. Below, the specific implementation steps of the embodiment of the present invention are introduced:
[0044] 1) Data acquisition: collect EEG signals in a resting state, and perform preprocessing for different environmental interference, hardware conditions and research purposes;
[0045] 2) Calculate multi-scale time series: multi-scale processing is performed on single-channel time series to obtain generalized multi-scale coarse-grained time series;
[0046] 3) Construct a multi-scale brain function network: at the same scale, use the amplitude correlation degree and phase correlation degree between channels as the quantification standard to calculate the weighted brain function network matrix;
[0047] 4) Construct a multi-scale convolutional neural network that can learn multi-scale brain function networks:...
PUM
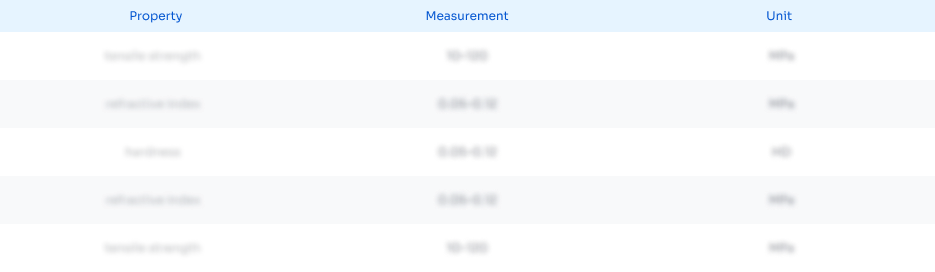
Abstract
Description
Claims
Application Information
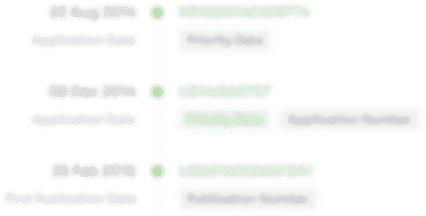
- R&D
- Intellectual Property
- Life Sciences
- Materials
- Tech Scout
- Unparalleled Data Quality
- Higher Quality Content
- 60% Fewer Hallucinations
Browse by: Latest US Patents, China's latest patents, Technical Efficacy Thesaurus, Application Domain, Technology Topic, Popular Technical Reports.
© 2025 PatSnap. All rights reserved.Legal|Privacy policy|Modern Slavery Act Transparency Statement|Sitemap|About US| Contact US: help@patsnap.com