Federated learning training data privacy enhancement method and system
A technology of learning and training and privacy, applied in the direction of digital transmission system, transmission system, electrical components, etc., to achieve the effect of increasing flexibility and scalability, ensuring security, and ensuring safety
- Summary
- Abstract
- Description
- Claims
- Application Information
AI Technical Summary
Problems solved by technology
Method used
Image
Examples
Embodiment 1
[0056] A method for enhancing the privacy of federated learning training data, applied to: n clients that do not trust each other, denoted as F i (i=1...n), and two semi-honest third-party servers, respectively denoted as the first server S and the second server S'. Among them, the server S' is configured to: be responsible for the communication between the client and the server S and the calculation inside S'; be responsible for storing the data uploaded by the client and various data in the calculation process; and store the public parameters sent by the server S PP. The server S is configured to: only interact with the server S'; be responsible for storing the data sent by the server S' and various data of the calculation process; be responsible for storing the public parameters and master keys generated by the BCP algorithm.
[0057] Such as Figure 1-2 As shown, the method specifically includes the following steps:
Embodiment 2
[0087] The purpose of this embodiment is to provide a system for enhancing the privacy of federated learning training data.
[0088] In order to achieve the above purpose, this embodiment provides a federated learning training data privacy enhancement system, including a first server, a second server and multiple clients participating in federated learning.
[0089] The first server generates public parameters and a master key, and sends the public parameters to the second server; if the encrypted model parameters and the corresponding public key are received, decrypt each blinded encrypted model parameter based on the master key, and pass weighted average to obtain the global model parameters, respectively adopting the public key of each client to encrypt the global model parameters, and sending to the second server;
[0090] The second server receives and stores public parameters; receives encrypted model parameters and corresponding public keys, and sends them to the first ...
Embodiment 3
[0093] The purpose of this embodiment is to provide a server.
[0094] The server is applied to federated learning, communicates with multiple clients participating in federated learning via another server, and is configured to:
[0095] Generate a public parameter and a master key, and send the public parameter to another server for downloading by multiple clients; wherein the public parameter is used for each client to generate its own public key;
[0096] Receiving encrypted model parameters and corresponding public keys from another server, wherein the encrypted model parameters are obtained by encrypting the local model parameters obtained by each client based on their respective public keys;
[0097] The encrypted model parameters are decrypted based on the master key, the global model parameters are obtained by weighted average, the global model parameters are encrypted with the public key of each client, and sent to each client through another server.
PUM
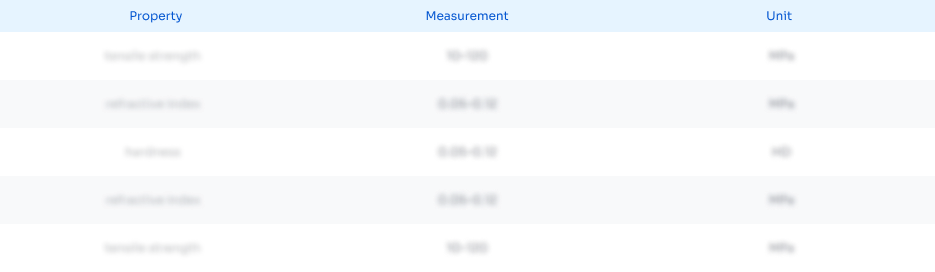
Abstract
Description
Claims
Application Information
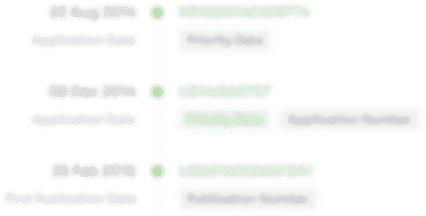
- R&D Engineer
- R&D Manager
- IP Professional
- Industry Leading Data Capabilities
- Powerful AI technology
- Patent DNA Extraction
Browse by: Latest US Patents, China's latest patents, Technical Efficacy Thesaurus, Application Domain, Technology Topic, Popular Technical Reports.
© 2024 PatSnap. All rights reserved.Legal|Privacy policy|Modern Slavery Act Transparency Statement|Sitemap|About US| Contact US: help@patsnap.com