Multi-modal fusion saliency detection method based on convolution block attention module
A detection method and multi-modal technology, applied in neural learning methods, character and pattern recognition, biological neural network models, etc., can solve the problem of unrepresentative image features, poor saliency prediction map effect, loss of image feature information, etc. problem, to achieve the effect of improving the efficiency of saliency detection, improving the efficiency and accuracy of detection, and improving the training results
- Summary
- Abstract
- Description
- Claims
- Application Information
AI Technical Summary
Problems solved by technology
Method used
Image
Examples
Embodiment Construction
[0039] The present invention will be described in further detail below in conjunction with the accompanying drawings and embodiments.
[0040] A saliency detection method based on multimodal fusion of convolutional block attention module proposed by the present invention, its overall realization block diagram is as follows figure 1 As shown, it includes two processes of training phase and testing phase;
[0041] The specific steps of the described training phase process are:
[0042] Step 1_1: Select the left viewpoint image, depth image and corresponding real human eye annotation image of N original stereoscopic images to form a training set, and record the left viewpoint image of the kth original stereoscopic image in the training set as The depth image of the original stereo image is denoted as The corresponding real human gaze is denoted as {G k (x, y)}; Since the left view image of the original stereo image, that is, the RGB color image has three channels, while the...
PUM
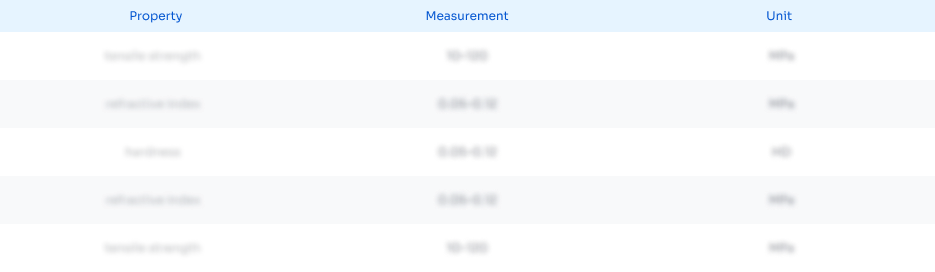
Abstract
Description
Claims
Application Information
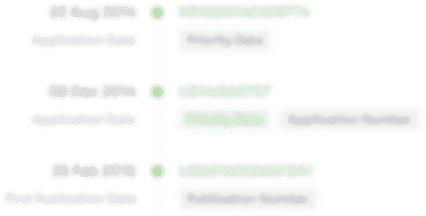
- R&D
- Intellectual Property
- Life Sciences
- Materials
- Tech Scout
- Unparalleled Data Quality
- Higher Quality Content
- 60% Fewer Hallucinations
Browse by: Latest US Patents, China's latest patents, Technical Efficacy Thesaurus, Application Domain, Technology Topic, Popular Technical Reports.
© 2025 PatSnap. All rights reserved.Legal|Privacy policy|Modern Slavery Act Transparency Statement|Sitemap|About US| Contact US: help@patsnap.com