Multi-domain image conversion method, system and medium based on conditional generative confrontation network
A conditional generation and image conversion technology, which is applied in the field of image style transfer and multi-modal conversion of medical images, can solve the problems of not being able to guarantee cars, not being able to generate pictures, etc., and achieve the effect of flexible training
- Summary
- Abstract
- Description
- Claims
- Application Information
AI Technical Summary
Problems solved by technology
Method used
Image
Examples
Embodiment Construction
[0059] The following will take the two-domain conversion of two modalities of x and y as an example to further describe in detail the multi-domain image conversion method and system of the present invention that can realize multi-modal conversion of medical images.
[0060] Such as figure 1 As shown, the implementation steps of the multi-domain image conversion method based on conditional generative confrontation network in this embodiment include:
[0061] 1) Input the original image x of the x mode to be converted, the original image y of the y mode;
[0062] 2) For the original image x, use a pre-trained condition extractor to produce x modal condition C x , using a pre-trained condition extractor for the original image y to produce the y-mode condition C y ;
[0063] 3) The original image x, original image y, x modal condition C x , y mode condition C y Input the pre-trained conditional generative adversarial network to get the corresponding image conversion result. ...
PUM
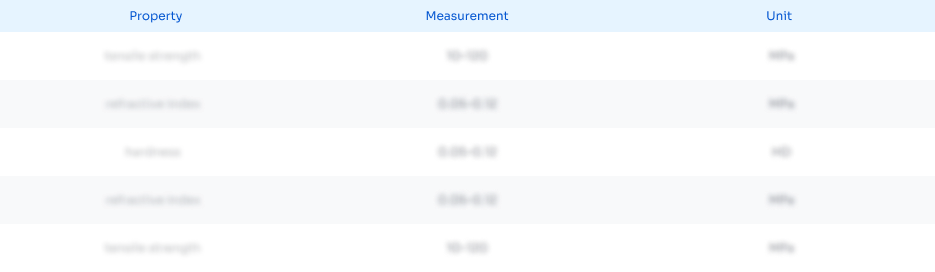
Abstract
Description
Claims
Application Information
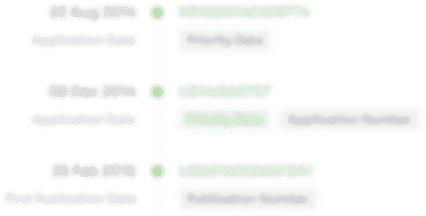
- R&D Engineer
- R&D Manager
- IP Professional
- Industry Leading Data Capabilities
- Powerful AI technology
- Patent DNA Extraction
Browse by: Latest US Patents, China's latest patents, Technical Efficacy Thesaurus, Application Domain, Technology Topic, Popular Technical Reports.
© 2024 PatSnap. All rights reserved.Legal|Privacy policy|Modern Slavery Act Transparency Statement|Sitemap|About US| Contact US: help@patsnap.com