Improved wavelet threshold denoising-based fault diagnosis method and improved wavelet threshold denoising-based fault diagnosis system
A wavelet threshold denoising and fault diagnosis technology, applied in instrument, calculation, character and pattern recognition, etc., can solve the problems of signal distortion, weak process variation analysis and detection ability, and inability to comprehensively use the soft threshold method. The effect of reliability
- Summary
- Abstract
- Description
- Claims
- Application Information
AI Technical Summary
Problems solved by technology
Method used
Image
Examples
Embodiment
[0113] In this embodiment, the TE process data set is used. The TE process was developed by Eastman Chemical Company to provide a realistic industrial process for evaluating process control and monitoring methods. TE process data has been commonly used in the field of fault diagnosis and process monitoring as a data source for comparing various methods. The TE process includes five main unit operations: reactor, condenser, cycle, compressor, separator, and stripper, and there are 21 types of failures in the TE process. There are 12 operating variables and 41 measured variables in this process, including 15 known disturbances and 6 working modes. The entire test data set includes a training set and a test set. The data in the dataset consists of 22 different simulated data, and each sample in the dataset has 52 observed variables. Each training set test sample represents a failure. Figure 4 is the TE process flow diagram.
[0114] The experimental environment is Windows 7...
Embodiment 2
[0121] Embodiment 2, this embodiment also provides a fault diagnosis system based on improved wavelet threshold denoising;
[0122] Fault diagnosis system based on improved wavelet threshold denoising, including:
[0123] An acquisition module configured to: acquire TE process (Tennessee Eastman Process, TE process is a process of real simulation of a chemical process) data to be diagnosed; standardize the acquired data;
[0124] A wavelet transform module configured to: perform wavelet transform decomposition on the standardized processed data, decompose into several layers, and obtain wavelet coefficients of each layer, and the wavelet coefficients include: low-frequency coefficients or high-frequency coefficients;
[0125] The noise reduction module is configured to: calculate the skewness coefficient and the kurtosis coefficient for the wavelet coefficient of each layer; judge whether the wavelet coefficient of the current layer conforms to the normal distribution accordin...
Embodiment 3
[0128] Embodiment 3. This embodiment also provides an electronic device, including a memory, a processor, and computer instructions stored in the memory and run on the processor. When the computer instructions are executed by the processor, the computer instructions in Embodiment 1 are completed. steps of the method described above.
PUM
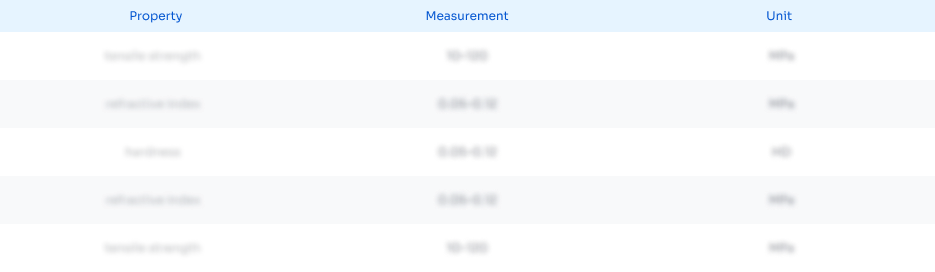
Abstract
Description
Claims
Application Information
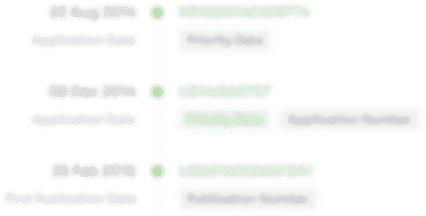
- R&D Engineer
- R&D Manager
- IP Professional
- Industry Leading Data Capabilities
- Powerful AI technology
- Patent DNA Extraction
Browse by: Latest US Patents, China's latest patents, Technical Efficacy Thesaurus, Application Domain, Technology Topic, Popular Technical Reports.
© 2024 PatSnap. All rights reserved.Legal|Privacy policy|Modern Slavery Act Transparency Statement|Sitemap|About US| Contact US: help@patsnap.com