Method for predicting atmospheric pollution conditions based on integrated gate recurrent unit neural network GRU
A neural network and air pollutant technology, applied in the field of air pollution prediction, can solve the problem of indistinct expression of the inherent characteristics of sequence data
- Summary
- Abstract
- Description
- Claims
- Application Information
AI Technical Summary
Problems solved by technology
Method used
Image
Examples
Embodiment 1
[0081] see Figure 1 to Figure 4 , based on the integrated gate recurrent unit neural network GRU to predict the method of air pollution, mainly including the following steps:
[0082] 1) Obtain the historical weather data set and preprocess the historical weather data set. The historical weather datasets include atmospheric pollutant datasets 11 (t) and meteorological datasets.
[0083] Further, the air pollutant data set s 11 (t) includes PM2.5 concentration data.
[0084] The meteorological data set includes date, dew point, temperature, atmospheric pressure, wind direction, wind speed, snowfall and / or rainfall.
[0085] Further, the main steps of preprocessing historical weather data are:
[0086] 1.1) Fill in 0 in the position of missing data in the historical weather data set.
[0087] 1.2) Delete the non-characteristic data columns in the historical weather data set. The non-characteristic data includes dates. Feature data includes air pollutant data, dew point,...
Embodiment 2
[0147] The present invention proposes a kind of method based on integrated gate recursive unit neural network (GRU) to predict air pollution situation, and this method comprises:
[0148] 1) Obtain historical weather data and preprocess the historical weather data; the obtained data comes from the historical weather data and air pollution index collected hourly by the US embassy in Beijing for 5 years from 2010 to 2014, the data set Including date, hourly PM2.5 concentration, dew point, temperature, wind direction, wind speed, snowfall and rainfall; the raw data is processed, and the scattered NA values in the data set are represented by 0, and the columns that are not features are deleted, such as time. Integer encoding of categorical features, such as wind direction. All data sets have a total of 43799*8 data.
[0149] 2) Divide the preprocessed historical weather data set into training data and test data according to a certain ratio;
[0150] In the preprocessed data...
PUM
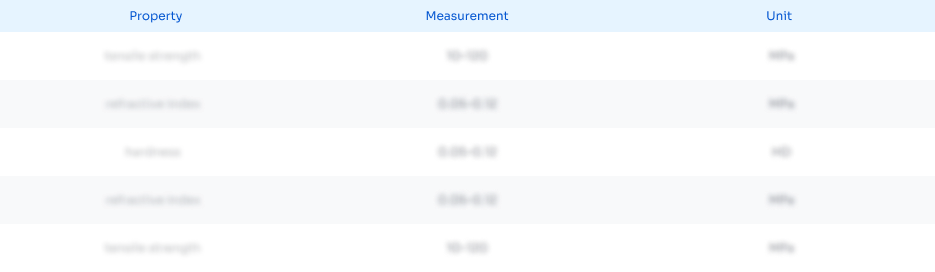
Abstract
Description
Claims
Application Information
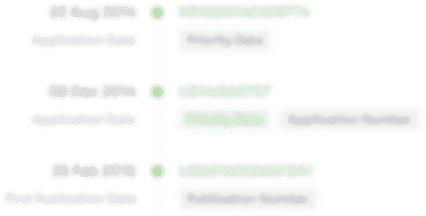
- Generate Ideas
- Intellectual Property
- Life Sciences
- Materials
- Tech Scout
- Unparalleled Data Quality
- Higher Quality Content
- 60% Fewer Hallucinations
Browse by: Latest US Patents, China's latest patents, Technical Efficacy Thesaurus, Application Domain, Technology Topic, Popular Technical Reports.
© 2025 PatSnap. All rights reserved.Legal|Privacy policy|Modern Slavery Act Transparency Statement|Sitemap|About US| Contact US: help@patsnap.com