Image semantic feature matching method based on geometric consistency
A semantic feature and feature matching technology, applied in the field of image feature matching and image semantic feature matching, can solve the problems of high dependency of feature descriptors, poor alignment ability, and poor alignment effect.
- Summary
- Abstract
- Description
- Claims
- Application Information
AI Technical Summary
Problems solved by technology
Method used
Image
Examples
Embodiment Construction
[0046] The process flow of the image semantic matching method based on geometric consistency proposed by the present invention is as follows: figure 1 As shown, the specific steps are as follows:
[0047] Step 1. Extract semantic features from the image to be matched. First, the image to be matched I A , I B Input the pre-trained VGG19 network for semantic feature extraction; then, specify the output of the relu1_1, relu2_1, relu3_1, relu4_1, and relu5_1 layers in the VGG19 network to construct the first, second, third, fourth, and fifth layers of the feature pyramid; finally, Use the Min-Max standardization strategy to quantify the salience of the semantic features of each layer of the feature pyramid, and construct the salient feature set Key_Points.
[0048] Step 2. Initialize semantic feature matching at the top level of the feature pyramid. According to the similar geometric information in the image to be matched, the apparent consistency constraint item, the orientat...
PUM
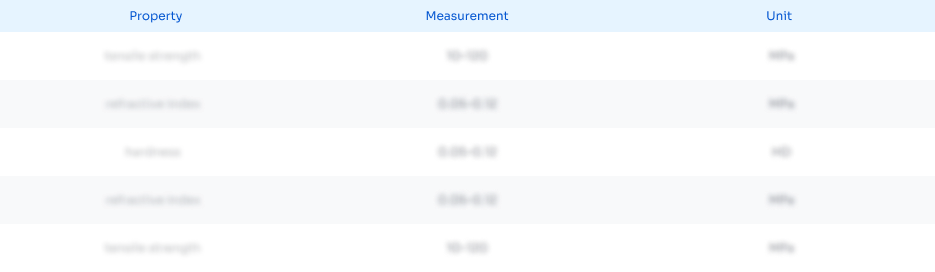
Abstract
Description
Claims
Application Information
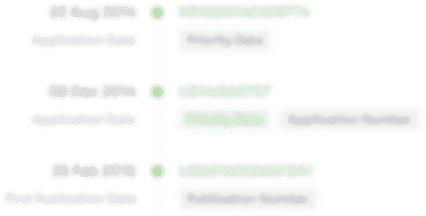
- R&D Engineer
- R&D Manager
- IP Professional
- Industry Leading Data Capabilities
- Powerful AI technology
- Patent DNA Extraction
Browse by: Latest US Patents, China's latest patents, Technical Efficacy Thesaurus, Application Domain, Technology Topic, Popular Technical Reports.
© 2024 PatSnap. All rights reserved.Legal|Privacy policy|Modern Slavery Act Transparency Statement|Sitemap|About US| Contact US: help@patsnap.com