Solar photovoltaic power generation prediction method based on TCN-LSTM
A solar photovoltaic and power generation forecasting technology, applied in forecasting, neural learning methods, instruments, etc., can solve problems such as the inability to accurately predict photovoltaic power generation
- Summary
- Abstract
- Description
- Claims
- Application Information
AI Technical Summary
Problems solved by technology
Method used
Image
Examples
Embodiment Construction
[0056] The present invention will be further described below in conjunction with the accompanying drawings and specific embodiments.
[0057] The present invention covers any alternatives, modifications, equivalent methods and schemes made on the spirit and scope of the present invention. In order to provide the public with a thorough understanding of the present invention, specific details are set forth in the following preferred embodiments of the present invention, but those skilled in the art can fully understand the present invention without the description of these details. In addition, for the sake of illustration, the drawings of the present invention are not completely drawn according to the actual scale, and are described here.
[0058] Such as figure 1 As shown, a kind of TCN-LSTM-based solar photovoltaic power generation prediction research of the present invention includes data set preprocessing, model building and performance evaluation, etc.; An acronym for Hy...
PUM
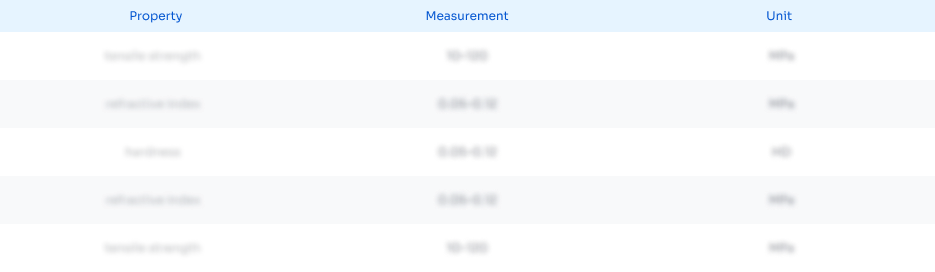
Abstract
Description
Claims
Application Information
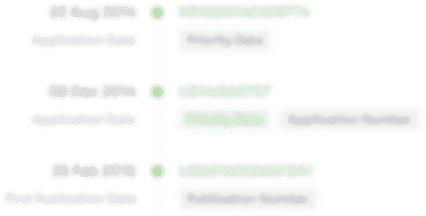
- R&D Engineer
- R&D Manager
- IP Professional
- Industry Leading Data Capabilities
- Powerful AI technology
- Patent DNA Extraction
Browse by: Latest US Patents, China's latest patents, Technical Efficacy Thesaurus, Application Domain, Technology Topic, Popular Technical Reports.
© 2024 PatSnap. All rights reserved.Legal|Privacy policy|Modern Slavery Act Transparency Statement|Sitemap|About US| Contact US: help@patsnap.com