Convolutional self-encoding fault monitoring method based on batch imaging
A convolutional self-encoding and fault monitoring technology, which is applied in the direction of kernel methods, neural learning methods, instruments, etc., can solve the problem of less fault monitoring applications, reduce modeling workload, reduce false positives, and improve accuracy
- Summary
- Abstract
- Description
- Claims
- Application Information
AI Technical Summary
Problems solved by technology
Method used
Image
Examples
Embodiment Construction
[0032] The Pensim penicillin fermentation simulation platform is a standard platform developed by Professor Cinar of the Illinois Institute of Technology and others for the evaluation of the effectiveness of fault monitoring in batch processes. In this experiment, a total of 10 process variables are collected. The variable names are shown in Table 1. The sampling interval is 1h. 50 normal batches are selected as training samples, and 2 faulty batches are used as test samples. Among them, the failure batch 1 is a step change of the ventilation rate with an amplitude of 1 at 200h, and the failure batch 2 is a ramp change of the stirring power at 200h with a slope of 0.003.
[0033] Table 1 Variables used to build the model
[0034]
[0035] Based on the above content, the present invention is applied to the above-mentioned fermentation process simulation platform, and the specific implementation steps are as follows:
[0036] A. Offline modeling phase:
[0037] 1): Collecti...
PUM
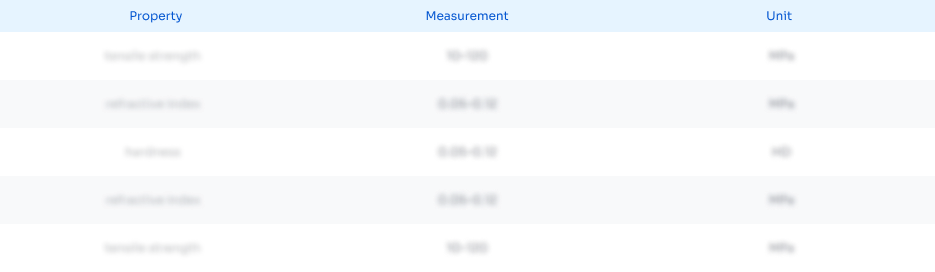
Abstract
Description
Claims
Application Information
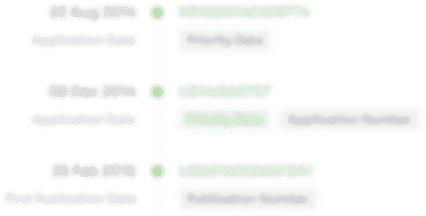
- R&D Engineer
- R&D Manager
- IP Professional
- Industry Leading Data Capabilities
- Powerful AI technology
- Patent DNA Extraction
Browse by: Latest US Patents, China's latest patents, Technical Efficacy Thesaurus, Application Domain, Technology Topic, Popular Technical Reports.
© 2024 PatSnap. All rights reserved.Legal|Privacy policy|Modern Slavery Act Transparency Statement|Sitemap|About US| Contact US: help@patsnap.com