Power distribution network line fault prediction method based on deep learning
A distribution network fault and deep learning technology, applied in neural learning methods, predictions, biological neural network models, etc., can solve problems such as complex structure of distribution network, affecting multiple lines, large cables, etc., to improve operation and maintenance Effects of Maintenance Efficiency and Power Supply Reliability
- Summary
- Abstract
- Description
- Claims
- Application Information
AI Technical Summary
Problems solved by technology
Method used
Examples
Embodiment Construction
[0018] The present invention will be further described below.
[0019] The causes of distribution network faults mainly include self-factors, natural factors and external factors.
[0020] Self-factors include operating factors and equipment factors. The operating factors that affect distribution network faults are mainly current, voltage and grid harmonics. The current and voltage in the distribution network are likely to cause problems such as heavy overload, low voltage and three-phase imbalance. , Heavy overload and low voltage not only make the distribution network equipment deviate from the rated working condition, but also cause the operating temperature of the equipment to rise; the three-phase imbalance will increase the energy consumption of the distribution network, and the overload of the heavy load phase will also cause equipment Temperature rise may affect the performance and life of distribution network equipment. Distribution network equipment factors are main...
PUM
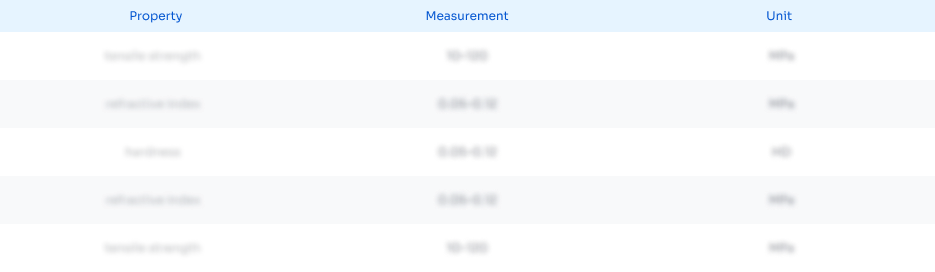
Abstract
Description
Claims
Application Information
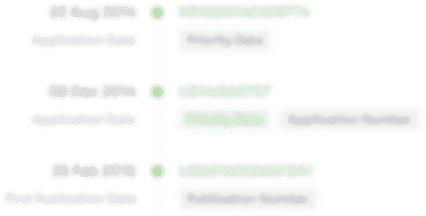
- R&D Engineer
- R&D Manager
- IP Professional
- Industry Leading Data Capabilities
- Powerful AI technology
- Patent DNA Extraction
Browse by: Latest US Patents, China's latest patents, Technical Efficacy Thesaurus, Application Domain, Technology Topic, Popular Technical Reports.
© 2024 PatSnap. All rights reserved.Legal|Privacy policy|Modern Slavery Act Transparency Statement|Sitemap|About US| Contact US: help@patsnap.com