Magnetotelluric deep neural network inversion method based on space mapping technology
A deep neural network and magnetotelluric technology, applied in neural learning methods, biological neural network models, neural architectures, etc., can solve problems such as sharp changes in resistivity values that do not conform to actual geological significance, increased calculations, and increased learning costs
- Summary
- Abstract
- Description
- Claims
- Application Information
AI Technical Summary
Problems solved by technology
Method used
Image
Examples
Embodiment 1
[0090] see Figure 1 to Figure 3 , a magnetotelluric deep neural network inversion method based on space mapping technology, mainly includes the following steps:
[0091] 1) Determine the detection area, that is, the layered geoelectric section.
[0092] 2) Establish geoelectric model sample set A 2 , the main steps are as follows:
[0093] 2.1) Establish a training sample set G based on the resistivity of each layer of geoelectric section, namely:
[0094]
[0095] In the formula, σ 0 and σ 1 Represent the minimum conductivity and maximum conductivity in the training sample set G, respectively. n is the set G capacity. When the number of ground section layers is M, the number of subsets of the training sample set is n M . i represents any sample.
[0096] 2.2) Use the conductivity constrained sampling strategy to simplify the training sample set G, and obtain the simplified training sample set A 1 . Simplify the training sample set A 1 The number of subsets is ...
Embodiment 2
[0170] A method for inversion of magnetotelluric deep neural network based on space mapping technology, mainly comprising the following steps:
[0171] 1) Determine the detection area, that is, the layered geoelectric section.
[0172] 2) Establish geoelectric model sample set A 2 , the main steps are as follows:
[0173] 2.1) Establish a training sample set G based on the resistivity of each layer of geoelectric section, namely:
[0174]
[0175] In the formula, σ 0 and σ 1 Represent the minimum conductivity and maximum conductivity in the training sample set G; n is the capacity of the set G; when the number of local electric section layers is M, the number of subsets of the training sample set is n M .
[0176] 2.2) Use the conductivity constrained sampling strategy to simplify the training sample set G, and obtain the simplified training sample set A 1 ; Simplify the training sample set A 1 The number of subsets is n×3 M-1 ;
[0177] The conductivity-constraine...
Embodiment 3
[0189] A method for inversion of magnetotelluric deep neural network based on space mapping technology, the main steps are shown in embodiment 2, wherein, the establishment of magnetotelluric forward modeling response data set A 3 The main steps are as follows:
[0190] 1) Using the magnetotelluric sounding method to calculate the orthogonal components of the electric field E and magnetic field H on the earth's surface.
[0191] 2) Based on the electric field E and magnetic field H, establish the magnetotelluric detection data set Z, namely:
[0192]
[0193] In the formula, Z is the impedance tensor used to characterize the electromagnetic field relationship. x and y represent two-dimensional coordinate directions. Among them, Z xx = 0, Z yy = 0, Z xy =-Z yx .
[0194] 3) Calculate the top surface impedance Z of the mth layer m ,which is:
[0195]
[0196] Among them, k m is the wavenumber of the mth layer. h m is the layer thickness of the mth layer. Z om...
PUM
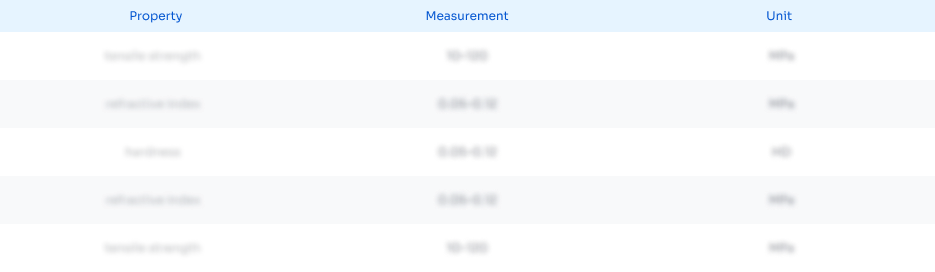
Abstract
Description
Claims
Application Information
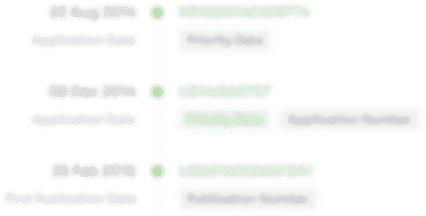
- R&D Engineer
- R&D Manager
- IP Professional
- Industry Leading Data Capabilities
- Powerful AI technology
- Patent DNA Extraction
Browse by: Latest US Patents, China's latest patents, Technical Efficacy Thesaurus, Application Domain, Technology Topic, Popular Technical Reports.
© 2024 PatSnap. All rights reserved.Legal|Privacy policy|Modern Slavery Act Transparency Statement|Sitemap|About US| Contact US: help@patsnap.com