Neural network acceleration system based on FPGA
A neural network and acceleration system technology, applied in the fields of artificial intelligence and electronics, can solve the problems of computing acceleration, increasing costs, consuming large resources and energy, etc., and achieve the effects of increasing inference speed, reducing power consumption, and increasing computing speed
- Summary
- Abstract
- Description
- Claims
- Application Information
AI Technical Summary
Problems solved by technology
Method used
Image
Examples
Embodiment Construction
[0017] The technical solution of the present invention will be specifically described below in conjunction with the accompanying drawings.
[0018] The present invention provides an FPGA-based neural network acceleration system. According to the natural parallelism of the convolutional neural network and the sparsity of the fully connected layer, the system reuses computing resources, parallelizes data and pipeline design, and utilizes fully connected The sparsity of the layer design sparse matrix multiplier greatly improves the operation speed and reduces the use of resources, so as to improve the inference speed without affecting the inference accuracy of the convolutional neural network. The system includes a data input module, a convolution processing module, a pooling module, a convolution control module, a non-zero detection module, a sparse matrix multiplier, and a classification output module; the convolution control module controls the data to be convoluted and the neu...
PUM
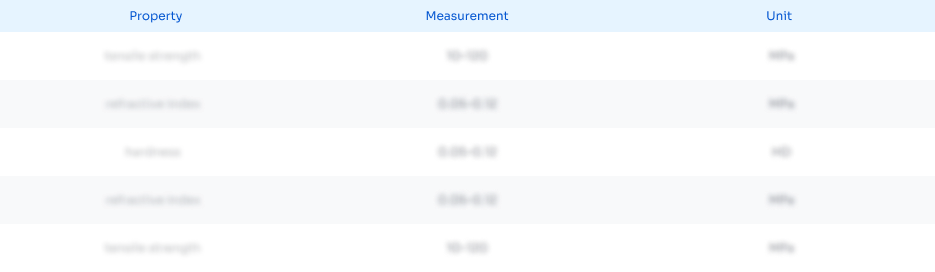
Abstract
Description
Claims
Application Information
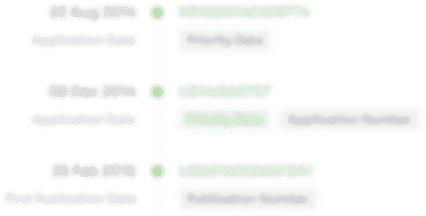
- Generate Ideas
- Intellectual Property
- Life Sciences
- Materials
- Tech Scout
- Unparalleled Data Quality
- Higher Quality Content
- 60% Fewer Hallucinations
Browse by: Latest US Patents, China's latest patents, Technical Efficacy Thesaurus, Application Domain, Technology Topic, Popular Technical Reports.
© 2025 PatSnap. All rights reserved.Legal|Privacy policy|Modern Slavery Act Transparency Statement|Sitemap|About US| Contact US: help@patsnap.com