Chip surface defect classification device and method based on generative adversarial network
A defect classification and generative technology, applied in biological neural network models, measurement devices, neural learning methods, etc., can solve the problem of difficult to meet the training data demand of deep convolutional neural network, can not effectively improve the classification performance of recognition models, defect pictures Issues such as limited quantity, to achieve the effect of improving classification accuracy, improving classification efficiency, and improving training accuracy
- Summary
- Abstract
- Description
- Claims
- Application Information
AI Technical Summary
Problems solved by technology
Method used
Image
Examples
Embodiment 1
[0054] The image processing module in the present invention includes a generative confrontation network. When both the generator and the judger are units based on the convolutional neural network, the input and output schematic diagram of the generator is shown in the attached image 3 As shown, the input and output schematic diagram of the judge is as attached Figure 4 As shown, the specific steps for the generator and the judger to generate a composite image in the image processing module are as follows:
[0055] T01: Input the random vector into the input layer of the generator, the size of the input layer of the generator is 100×1; in this embodiment, the size of the input layer of the generator is set according to the defect type of the synthesized image, and can also be other values;
[0056] T02: The synthetic image output by the input layer of the generator is input to the first convolutional layer GC1 of the generator. The size of the first convolutional layer GC1 of...
PUM
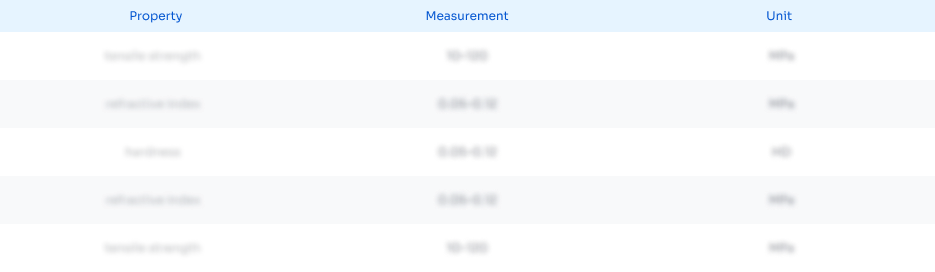
Abstract
Description
Claims
Application Information
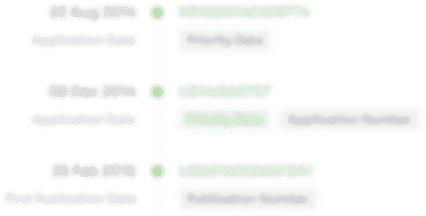
- R&D Engineer
- R&D Manager
- IP Professional
- Industry Leading Data Capabilities
- Powerful AI technology
- Patent DNA Extraction
Browse by: Latest US Patents, China's latest patents, Technical Efficacy Thesaurus, Application Domain, Technology Topic, Popular Technical Reports.
© 2024 PatSnap. All rights reserved.Legal|Privacy policy|Modern Slavery Act Transparency Statement|Sitemap|About US| Contact US: help@patsnap.com