Cascade hydropower station generating capacity prediction method based on long-short-term memory network
A technology of long-term short-term memory and forecasting method, which is applied in the field of power generation forecasting of cascade hydropower stations based on long-term short-term memory network. It can solve the problems of not considering the long-term dependence of power generation time series and the difficulty of dispatching plan preparation, so as to improve the generalization Capability and stability, the effect of improving the fitting accuracy
- Summary
- Abstract
- Description
- Claims
- Application Information
AI Technical Summary
Problems solved by technology
Method used
Image
Examples
Embodiment Construction
[0058] Such as figure 1 As shown, the method for forecasting the power generation of cascade hydropower stations based on the long-term short-term memory network includes the following steps,
[0059] Step 1: For the data preprocessing of feature engineering, use the unit root test method to test the stationarity of the hydropower generation time series. When the sequence does not have a unit root, the sequence is considered to be stationary; otherwise, the sequence is considered to be a non-stationary time series ;
[0060] Step 2: Perform correlation test on the time series of power generation, calculate Pearson correlation coefficient and Spearman correlation coefficient, and select highly correlated power generation influencing factors;
[0061] Step 3: Convert the power generation time series data into supervised learning data;
[0062] Step 4: Establish a power generation prediction model based on the long-term short-term memory network;
[0063] Step 5: Perform integ...
PUM
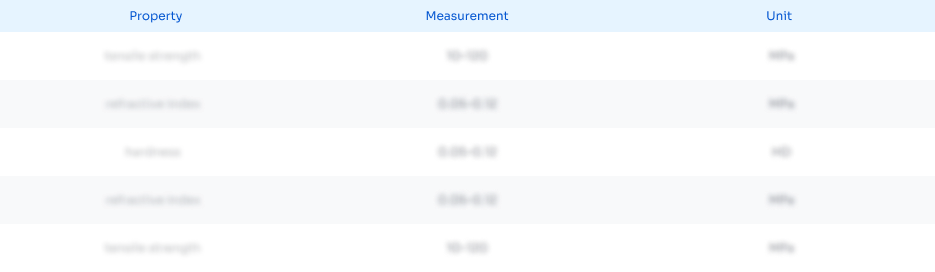
Abstract
Description
Claims
Application Information
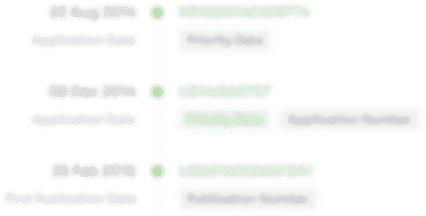
- R&D Engineer
- R&D Manager
- IP Professional
- Industry Leading Data Capabilities
- Powerful AI technology
- Patent DNA Extraction
Browse by: Latest US Patents, China's latest patents, Technical Efficacy Thesaurus, Application Domain, Technology Topic, Popular Technical Reports.
© 2024 PatSnap. All rights reserved.Legal|Privacy policy|Modern Slavery Act Transparency Statement|Sitemap|About US| Contact US: help@patsnap.com