Compressed sampling sensing matrix disturbance optimization model construction method based on statistical learning
A sensor matrix and compressed sampling technology, applied in the field of signal processing, can solve problems such as measurement disturbance and result deviation, achieve accurate analysis and improve accuracy
- Summary
- Abstract
- Description
- Claims
- Application Information
AI Technical Summary
Problems solved by technology
Method used
Image
Examples
Embodiment
[0027] Such as figure 1 As shown, a method for constructing a perturbation optimization model for compressed sampling sensing matrix based on statistical learning includes the following steps:
[0028] S1. Set a mathematical model with disturbance, the mathematical model with disturbance includes a measurement matrix mathematical model Φ with disturbance and a sparse matrix mathematical model Ψ with disturbance;
[0029] S2, obtain the disturbance mathematical model Α of sensing matrix according to the measurement matrix mathematical model Φ with disturbance and the sparse matrix mathematical model Ψ with disturbance, its formula is A=ΦΨ;
[0030] S3, obtain the sparse signal model of compressed sensing according to the perturbation mathematical model A of the sensing matrix Among them, n is additive Gaussian white noise, θ is a sparse vector, and y is an observed data vector;
[0031] S4. Establish a robust compressed sensing optimization function according to the sparse v...
PUM
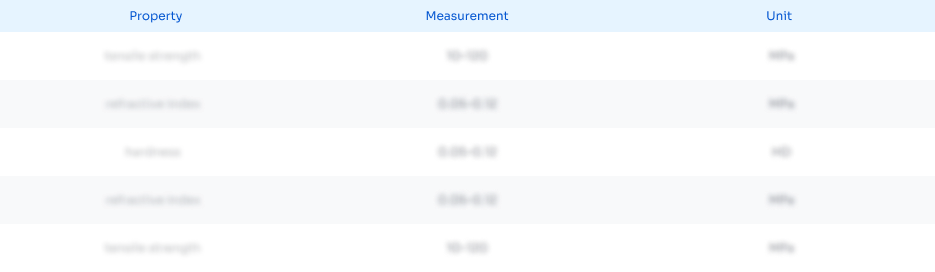
Abstract
Description
Claims
Application Information
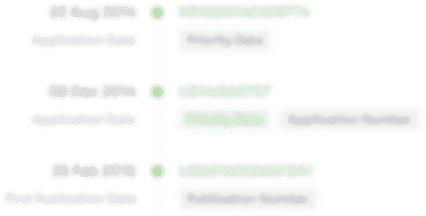
- R&D Engineer
- R&D Manager
- IP Professional
- Industry Leading Data Capabilities
- Powerful AI technology
- Patent DNA Extraction
Browse by: Latest US Patents, China's latest patents, Technical Efficacy Thesaurus, Application Domain, Technology Topic, Popular Technical Reports.
© 2024 PatSnap. All rights reserved.Legal|Privacy policy|Modern Slavery Act Transparency Statement|Sitemap|About US| Contact US: help@patsnap.com