Gastric mucosa cleanliness evaluation method and system based on deep learning
A technology of deep learning and evaluation methods, applied in image data processing, instruments, calculations, etc., can solve the problems of increasing misdiagnosis, missed diagnosis, low quality of gastroscope preparation, poor cleanliness of gastric mucosa, etc.
- Summary
- Abstract
- Description
- Claims
- Application Information
AI Technical Summary
Problems solved by technology
Method used
Image
Examples
Embodiment 1
[0048] Such as figure 1 As shown, this embodiment discloses a method for evaluating the cleanliness of gastric mucosa based on deep learning, comprising the following steps:
[0049] Step 1: Construct the evaluation model of gastric mucosal cleanliness. The step 1 specifically includes:
[0050] Step 1.1: Obtain the gastroscope training image set.
[0051] Such as figure 2 As shown, the patient’s gastroscope is projected to the UI interface of the gastric mucosa cleanliness evaluation system by using a video capture card to obtain the frame rate and resolution of the video source of the gastroscope, and based on the video capture card development interface, real-time acquisition of a single frame image or per minute Collect n frames (5-10 frames are recommended).
[0052] Step 1.2: Establish an evaluation system for gastric mucosal cleanliness.
[0053] As an implementation, the grades and scores in the gastric mucosal cleanliness evaluation system are all formulated by ...
Embodiment approach
[0072] As an implementation, the gastric mucosa cleanliness evaluation model construction method includes:
[0073] Based on the same training set, verification set, and test set, AlexNet, SqueezeNet, MobileNet V1-V3, ShuffleNetV1-V2, and Xception deep convolutional networks were trained simultaneously, and group convolution was used in the network (group convolution in AlexNet, not only Optimize the speed of model training, and improve the diversity of features extracted by convolution), depth separable convolution (MobileNet V1-V2, depth separable convolution is used in Xception, while reducing the amount of training parameters, Increased inference speed), residual block (MobileNet V2, residual block is used in Xception, which avoids gradient explosion and enhances multi-level feature extraction), NAS (MobileNet V3 uses NAS to select the network independently) The optimal network structure ensures the prediction accuracy while reducing the size of the model), File Module (in...
Embodiment 2
[0087] The purpose of this embodiment is to provide a gastric mucosa cleanliness evaluation system based on deep learning.
[0088] In order to achieve the above purpose, this embodiment provides a gastric mucosa cleanliness evaluation system based on deep learning, including:
[0089] The training data set acquisition module obtains the gastroscope training image set;
[0090] The scoring and labeling module marks and scores each training image according to the preset gastroscope cleanliness evaluation system;
[0091] The evaluation model building block uses the training image set to train the gastric mucosa cleanliness evaluation model based on the deep learning network;
[0092] Real-time data acquisition module, real-time acquisition of gastroscope video frames;
[0093] The cleanliness evaluation module is based on the pre-built gastric mucosal cleanliness evaluation model, and performs cleanliness evaluation frame by frame.
PUM
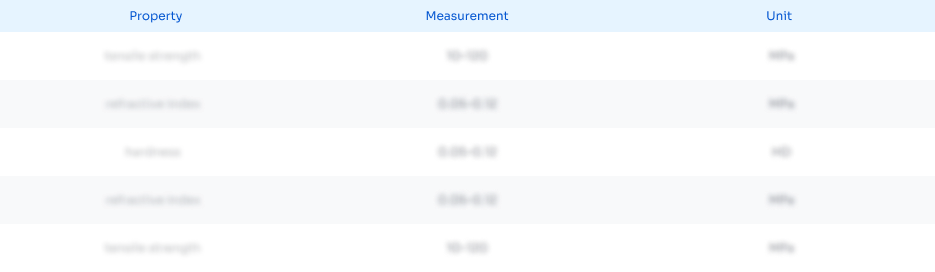
Abstract
Description
Claims
Application Information
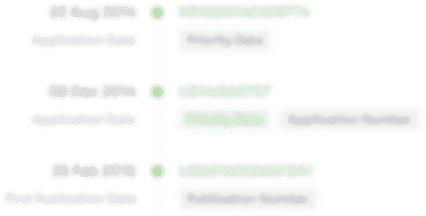
- R&D Engineer
- R&D Manager
- IP Professional
- Industry Leading Data Capabilities
- Powerful AI technology
- Patent DNA Extraction
Browse by: Latest US Patents, China's latest patents, Technical Efficacy Thesaurus, Application Domain, Technology Topic, Popular Technical Reports.
© 2024 PatSnap. All rights reserved.Legal|Privacy policy|Modern Slavery Act Transparency Statement|Sitemap|About US| Contact US: help@patsnap.com